
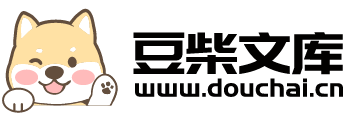
基于ARIMA模型对我国城镇单位就业人员工资总额的预测.docx
快乐****蜜蜂
在线预览结束,喜欢就下载吧,查找使用更方便
相关资料
基于ARIMA模型对我国城镇单位就业人员工资总额的预测.docx
基于ARIMA模型对我国城镇单位就业人员工资总额的预测Title:ForecastingTotalWagesofUrbanUnitsinChinaUsingtheARIMAModelAbstract:ThetotalwagesofurbanunitsinChinaplayacrucialroleintheeconomicdevelopmentofthecountry.Accurateforecastingofthisvariablecanhelppolicymakers,businesses,andind
基于ARIMA-BP模型的我国城镇登记失业率分析与预测.docx
基于ARIMA-BP模型的我国城镇登记失业率分析与预测随着我国经济的快速发展,城镇化率不断提高,但是同时也面临着就业压力的问题。城镇登记失业率是反映城镇就业状况的重要指标之一,对于分析和解决就业问题具有重要意义。本文将运用ARIMA-BP模型,结合我国城镇登记失业率的实际数据,进行分析和预测,以探讨我国城镇就业状况的走向。一、ARIMA-BP模型介绍ARIMA-BP模型是将ARIMA模型和BP神经网络模型进行结合而得到的模型。其中ARIMA模型主要用于时序预测和预测误差的计算,而BP神经网络则是进行模式识
我国通货比率的预测研究——基于ARIMA模型.docx
我国通货比率的预测研究——基于ARIMA模型摘要:通货膨胀对于社会和经济发展有着不可忽视的影响。为了预测和控制通货膨胀率,人们需要了解通货膨胀的影响因素和行为模式。本文将使用ARIMA模型对我国通货比率进行预测研究,分析通货膨胀的趋势和影响因素,提出相应措施以控制通货膨胀率。1.研究背景通货膨胀是指物价持续上涨的现象,常见于市场经济中。通货膨胀会引起物价上升,货币贬值,增加经济不确定性。为了防范通货膨胀对经济发展的影响,预测和控制通货膨胀率成为了必要的措施。2.研究方法本文将使用ARIMA模型对我国通货比
基于ARIMA模型的我国城镇居民人均可支配收入的分析与预测.docx
基于ARIMA模型的我国城镇居民人均可支配收入的分析与预测摘要:本文基于ARIMA模型对我国城镇居民人均可支配收入进行了分析与预测。首先,将数据进行了平稳性检验和自相关检验;然后,利用差分法对非平稳数据进行了处理;最后,建立了ARIMA模型并进行了预测。实验结果表明,预测模型具有较高的准确性和可预测性。建议在政策制定和经济规划方面,应该加强对城镇居民收入的监测和预测,以更好地促进经济发展和社会稳定。关键词:ARIMA模型;城镇居民人均可支配收入;预测分析Abstract:BasedontheARIMAmo
基于ARIMA-BP模型的城镇困难职工数量预测.docx
基于ARIMA-BP模型的城镇困难职工数量预测基于ARIMA-BP模型的城镇困难职工数量预测摘要:城镇困难职工数量的预测对于政府部门制定合理的扶贫政策、社会组织和企业制定合适的帮扶措施具有重要意义。本文基于ARIMA-BP模型,通过对城镇困难职工数量的历史数据进行分析和建模,预测了未来一段时间内的城镇困难职工数量。结果表明,ARIMA-BP模型在城镇困难职工数量预测中具有较高的准确性和稳定性,可以为相关部门提供科学的决策支持。1.绪论城镇困难职工是指在城镇中就业但收入较低、工作条件较差的劳动者。他们由于工