
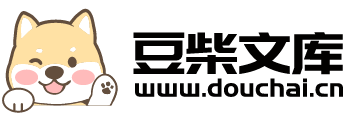
基于多任务卷积神经网络人脸检测网络的优化加速方法.docx
快乐****蜜蜂
在线预览结束,喜欢就下载吧,查找使用更方便
相关资料
基于多任务卷积神经网络人脸检测网络的优化加速方法.docx
基于多任务卷积神经网络人脸检测网络的优化加速方法Title:OptimizationandAccelerationofMulti-TaskConvolutionalNeuralNetworkforFaceDetectionAbstract:Facedetectionisafundamentaltaskincomputervisionwithnumerousapplicationssuchasfacialrecognition,emotionanalysis,andsurveillance.Multi-ta
基于多任务神经网络的人脸状态检测方法.pdf
本发明公开了一种基于多任务神经网络的人脸状态检测方法,包括图像预处理:将待检测图像输入至Dlib库中,在Dlib库中通过人脸定位算法,结合宽容度算法输出预处理后图像,预处理后图像中包含裁决后的完整的人脸图像;多任务神经网络输出面部特征点与头部姿态参数:构建基于面部特征点检测和头部姿态参数检测的多任务神经网络,所述多任务神经网络包括核心网络和子网络1、子网络2,子网络1、子网络2共享核心网络,子网络1用于实现面部特征点检测,子网络2用于头部姿态参数检测;人脸状态监测。本发明将面部特征点检测与头部姿态参数检测
基于多任务神经网络的人脸状态检测方法.pdf
本发明公开了一种基于多任务神经网络的人脸状态检测方法,包括图像预处理:将待检测图像输入至Dlib库中,在Dlib库中通过人脸定位算法,结合宽容度算法输出预处理后图像,预处理后图像中包含裁决后的完整的人脸图像;多任务神经网络输出面部特征点与头部姿态参数:构建基于面部特征点检测和头部姿态参数检测的多任务神经网络,所述多任务神经网络包括核心网络和子网络1、子网络2,子网络1、子网络2共享核心网络,子网络1用于实现面部特征点检测,子网络2用于头部姿态参数检测;人脸状态监测。本发明将面部特征点检测与头部姿态参数检测
基于多任务卷积神经网络的实时人眼检测方法.pptx
添加副标题目录PART01PART02卷积神经网络的基本结构多任务学习的基本概念多任务卷积神经网络在人眼检测中的应用实时性在人眼检测中的重要性PART03数据集的准备训练过程损失函数的定义优化算法的选择PART04模型结构的优化正则化技术的应用模型训练过程中的过拟合问题模型泛化能力的提高PART05人眼检测的流程人眼检测的精度和速度的平衡人眼检测的准确性和实时性的关系人眼检测的鲁棒性分析PART06实验设置和数据集性能评估指标实验结果和分析结果的可视化展示感谢您的观看
基于多任务深度卷积神经网络的人脸面瘫表情识别方法.docx
基于多任务深度卷积神经网络的人脸面瘫表情识别方法基于多任务深度卷积神经网络的人脸面瘫表情识别方法摘要:人脸表情是人与人之间交流和情感传递的重要方式之一。然而,面瘫患者的表情受限,往往无法有效地表达情感。为了帮助面瘫患者更好地进行情感交流,本文提出了一种基于多任务深度卷积神经网络的人脸面瘫表情识别方法。该方法利用深度卷积神经网络从面瘫患者的面部图像中提取特征,并利用多任务学习的方法同时进行表情识别和面瘫程度估计。实验结果表明,该方法在人脸面瘫表情识别任务中具有较高的准确率和稳定性,能够有效地帮助面瘫患者表达