
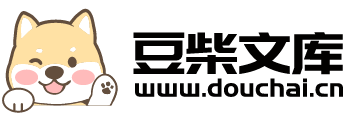
贝叶斯分析方法研究.docx
小新****ou
亲,该文档总共24页,到这已经超出免费预览范围,如果喜欢就直接下载吧~
相关资料
贝叶斯分析方法研究.docx
贝叶斯分析方法研究贝叶斯分析方法研究摘要机器学习作为一门人工智能的科学自20世纪50年代被提出以来,经过人们的不断研究,已形成了一套科学系统的理论。机器学习中一个很重要的步骤是特征的选择与提取,原始特征的数量可能很大,或者说样本处在一个高维空间中,我们需要找到一个合理的方法,降低特征数量的同时,尽量减少原特征中包含信息的损失,因子分析法就是这样一种降维的方法。然而由于因子分析模型中存在不可观测的隐变量,普通的极大似然法很难得到其参数的估计。贝叶斯理论提供了一种计算各变量后验概率的方法,这种方法基于假设的先
预案分析的贝叶斯网络方法.docx
预案分析的贝叶斯网络方法贝叶斯网络是一种基于概率论的图模型,它利用概率分布来描述随机变量之间的关系,并且通过这种关系来进行推理、预测和决策。贝叶斯网络已经被广泛用于风险评估和安全管理,特别是在预案分析领域的应用较为突出。本文将介绍贝叶斯网络在预案分析领域的应用,并深入探讨其原理和优缺点。一、贝叶斯网络在预案分析中的应用贝叶斯网络可以用于预测和识别复杂事件的可能性,因此在预案分析中非常重要。预案分析旨在识别可能的灾难风险,评估各种情况的应对措施,并提出应对策略,以应对可能发生的灾难情况。而贝叶斯网络则可以帮
贝叶斯分析.docx
编号:时间:2021年x月x日书山有路勤为径,学海无涯苦作舟页码:第页共NUMPAGES19页第PAGE\*MERGEFORMAT19页共NUMPAGES\*MERGEFORMAT19页第四章贝叶斯分析BayeseanAnalysis§4.0引言一、决策问题的表格表示——损失矩阵对无观察(No-data)问题a=δ可用表格(损失矩阵)替代决策树来描述决策问题的后果(损失):……π()…π()…π()或π()…π()…π()……损失矩阵直观、运算方便二、决策原则通常,要根
贝叶斯分析.docx
编号:时间:2021年x月x日书山有路勤为径学海无涯苦作舟页码:第四章贝叶斯分析BayeseanAnalysis§4.0引言一、决策问题的表格表示——损失矩阵对无观察(No-data)问题a=δ可用表格(损失矩阵)替代决策树来描述决策问题的后果(损失):……π()…π()…π()或π()…π()…π()……损失矩阵直观、运算方便二、决策原则通常要根据某种原则来选择决策规则δ使结果最优(或满意)这种原则就叫决策原则贝叶斯分析的
风险分析中的稳健贝叶斯方法.docx
风险分析中的稳健贝叶斯方法随着现代社会的发展,风险已经成为人们日常生活中难以避免的一部分。风险分析成为了对任何企业、组织和政府而言都是必不可少的流程。风险分析的目标是识别可能存在的风险,评估其潜在影响并制定合适的应对措施。而稳健贝叶斯方法则是一种重要的风险分析方法。稳健贝叶斯方法是贝叶斯分析的一种变体,它是一种能够处理许多不确定因素的灵活而更加统计强大的方法,并且能够更好地适应各种数据分布和不确定性。与传统的贝叶斯分析相比,稳健贝叶斯方法更加关注模型假设的鲁棒性,即使模型的假设不满足,也可以提供更加鲁棒的