
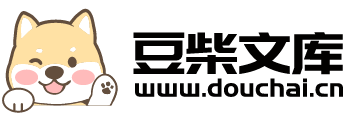
基于主题模型的多示例多标记学习方法.docx
康佳****文库
亲,该文档总共17页,到这已经超出免费预览范围,如果喜欢就直接下载吧~
相关资料
基于主题模型的多示例多标记学习方法.docx
基于主题模型的多示例多标记学习方法摘要:针对现有的大部分多示例多标记(MIML)算法都没有考虑如何更好地表示对象特征这一问题将概率潜在语义分析(PLSA)模型和神经网络(NN)相结合提出了基于主题模型的多示例多标记学习方法。算法通过概率潜在语义分析模型学习到所有训练样本的潜在主题分布该过程是一个特征学习的过程用于学习到更好的特征表达用学习到的每个样本的潜在主题分布作为输入来训练神经网络。当给定一个测试样本时学习测试样本的潜在主题分布将学习到的潜在主题分布输入到训练好的神经
基于主题模型的多示例多标记学习方法.docx
基于主题模型的多示例多标记学习方法摘要:针对现有的大部分多示例多标记(MIML)算法都没有考虑如何更好地表示对象特征这一问题将概率潜在语义分析(PLSA)模型和神经网络(NN)相结合提出了基于主题模型的多示例多标记学习方法。算法通过概率潜在语义分析模型学习到所有训练样本的潜在主题分布该过程是一个特征学习的过程用于学习到更好的特征表达用学习到的每个样本的潜在主题分布作为输入来训练神经网络。当给定一个测试样本时学习测试样本的潜在主题分布将学习到的潜在主题分布输入到训练好的神经
基于正示例与多示例多标记的图像检索.docx
基于正示例与多示例多标记的图像检索引言:近年来,随着数字图像数据规模的快速增长,基于图像检索的需求变得越来越迫切。图像检索技术可以方便地检索出与用户需求相匹配的图像,为用户提供更加便捷的图像搜索服务。同时,由于数字图像的多样性和复杂性,如何有效地进行图像检索也成为了一个重要的研究方向。本文旨在介绍几种不同的图像检索方法,并比较各个方法的优缺点。正文:一、传统的基于特征向量的图像检索方法在传统的基于特征向量的图像检索方法中,首先需要将每张图像都转换成一个特征向量,然后通过计算特征向量之间的相似度来进行图像检
基于正示例与多示例多标记的图像检索的中期报告.docx
基于正示例与多示例多标记的图像检索的中期报告本次中期报告主要介绍正示例与多示例多标记的图像检索问题及目前的研究进展。一、研究背景随着互联网时代的到来,人们每天都会使用大量的图像数据进行工作和娱乐,如何高效、准确地检索图像成为了一个重要问题。传统的图像检索通常基于单标记,即每张图像只有一个标记或一个标记列表。然而,现实中的图像往往是多标记的,且每个标记可能有不同的权重和重要性。因此,多标记图像检索问题被提出,其中一种特殊的情况是多示例多标记图像检索问题。这种情况下每个图像既有正示例标记,也有负示例标记。二、
多示例多标记哈希学习方法研究综述报告.docx
多示例多标记哈希学习方法研究综述报告多示例多标记哈希学习是一种用于处理多标记分类问题的机器学习方法。该方法由多示例学习(MIL)和哈希学习(HL)两个部分组成,能够有效地提高多标记分类问题的处理效率。在多标记分类问题中,一个对象可以被赋予多个标记,例如,在一个图像数据库中,一张图片可以有多个标签,比如“狗”、“植物”、“自然风景”等等。如果每一张图片都需要手动标注,那么工作量将是非常大的。因此,多标记分类问题在实际中具有很高的价值。多示例学习是一种解决多标记分类问题的方法,它在训练集中用一组示例来表示一个