
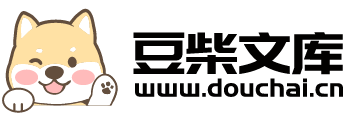
基于交替方向乘子法的非光滑损失坐标优化算法.docx
书生****22
亲,该文档总共11页,到这已经超出免费预览范围,如果喜欢就直接下载吧~
相关资料
基于交替方向乘子法的非光滑损失坐标优化算法.docx
基于交替方向乘子法的非光滑损失坐标优化算法交替方向乘子法(AlternatingDirectionMethodofMultipliers,ADMM)是一种经典的优化算法,可用于求解非光滑损失坐标优化问题。本文主要介绍ADMM的基本原理和步骤,并详细讨论它在求解非光滑损失坐标优化问题中的应用。一、引言在实际应用中,经常会遇到非光滑的损失函数,如L1损失函数和Huber损失函数等。这类损失函数难以直接优化,需要采用特殊的算法进行求解。ADMM是一种可行的选择,它通过将原始问题转化为一系列子问题,利用迭代的方式
基于交替方向乘子法的非光滑损失坐标优化算法.docx
基于交替方向乘子法的非光滑损失坐标优化算法文章编号:10019081(2013)07191205doi:10.11772/j.issn.10019081.2013.07.1912摘要:交替方向乘子法(ADMM)在机器学习问题中已有一些实际应用。针对大规模数据的处理和非光滑损失凸优化问题将镜面下降方法引入原ADMM批处理算法得到了一种新的改进算法并在此基础上提出了一种求解非光滑损失凸优化问题的坐标优化算法。该算法具有操作简单、计算高效的特点。
基于交替方向乘子法的非光滑损失坐标优化算法.docx
基于交替方向乘子法的非光滑损失坐标优化算法文章编号:10019081(2013)07191205doi:10.11772/j.issn.10019081.2013.07.1912摘要:交替方向乘子法(ADMM)在机器学习问题中已有一些实际应用。针对大规模数据的处理和非光滑损失凸优化问题将镜面下降方法引入原ADMM批处理算法得到了一种新的改进算法并在此基础上提出了一种求解非光滑损失凸优化问题的坐标优化算法。该算法具有操作简单、计算高效的特点。
基于交替方向乘子法的分布式优化算法研究.docx
基于交替方向乘子法的分布式优化算法研究基于交替方向乘子法的分布式优化算法研究摘要:随着大数据和分布式计算的普及和发展,分布式优化算法成为解决大规模优化问题的重要方法之一。交替方向乘子法是一种基于分解和协同的分布式优化方法,通过将原优化问题转化为等价的次优化问题,并利用多个节点之间的协作求解,能够有效地解决大规模问题。本文主要研究了基于交替方向乘子法的分布式优化算法,探讨了其原理、应用和优势,并通过案例分析进行实证研究,验证了算法的有效性和性能。1.引言分布式优化算法是一种将原优化问题分解为多个子问题,然后
非凸非光滑分块优化问题Bregman乘子交替方向法收敛性分析的任务书.docx
非凸非光滑分块优化问题Bregman乘子交替方向法收敛性分析的任务书1.研究背景与意义在现实生活中,许多问题都可以被建模为一个非凸非光滑的分块优化问题。例如,在机器学习中,我们需要对一个大规模的数据集进行分类或者回归,这就可以被建模为一个非凸非光滑的优化问题。这类问题的优化难度很高,存在多个局部最优解,难以找到全局最优解。因此,研究如何高效地求解这类问题具有重要的理论意义和实际应用价值。Bregman乘子交替方向法是一种求解非凸分块优化问题的有效方法。在一些实际问题中,该方法能够比其他传统方法更快地达到最