
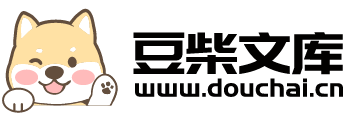
改进的粒子群优化算法用于方向图阵列综合.docx
快乐****蜜蜂
在线预览结束,喜欢就下载吧,查找使用更方便
相关资料
改进的粒子群优化算法用于方向图阵列综合.docx
改进的粒子群优化算法用于方向图阵列综合Title:ImprovedParticleSwarmOptimizationforDirectionalArraySynthesisAbstract:Thedirectionalarraysynthesisprobleminvolvestheoptimalarrangementandconfigurationofantennaelementsinordertoachievedesiredradiationpatterns.Inrecentyears,Particle
改进布谷鸟算法用于阵列天线方向图优化.docx
改进布谷鸟算法用于阵列天线方向图优化布谷鸟算法(BatAlgorithm,BA)是一种模拟鸟类群体行为的启发式算法,最初由Yang等人在2010年提出。该算法模拟了蝙蝠寻找食物的行为,通过调整频率和脉冲率来探索空间中的最佳解。由于其简单性和高效性,布谷鸟算法已在许多领域得到广泛应用,包括优化问题、模式识别和机器学习。本论文旨在改进布谷鸟算法,以优化阵列天线的方向图。首先,介绍阵列天线的基本原理和方向图优化问题。阵列天线由多个天线元素组成,可以控制信号的辐射和接收方向。方向图优化问题的目标是找到一组天线权重
基于二分粒子群优化的阵列天线方向图综合.docx
基于二分粒子群优化的阵列天线方向图综合基于二分粒子群优化的阵列天线方向图综合摘要:随着通信技术的迅速发展,阵列天线在无线通信系统中扮演着重要的角色。阵列天线的优化方向图是提高无线通信系统性能的关键因素之一。在本论文中,我们提出了一种基于二分粒子群优化的阵列天线方向图综合算法。该算法将天线方向图综合问题转化为一个离散优化问题,并通过引入二分法来加速算法的收敛速度。通过实验验证,我们的算法在不同实验设置下均取得了较好的性能,并能够快速收敛。关键词:阵列天线;方向图综合;二分粒子群优化;收敛速度引言:阵列天线是
基于改进遗传算法的圆阵列方向图联合优化.docx
基于改进遗传算法的圆阵列方向图联合优化基于改进遗传算法的圆阵列方向图联合优化摘要随着通信技术的发展和应用的广泛,圆阵列已成为一种重要的无线通信天线结构。优化圆阵列的方向图是提高无线通信系统性能的关键因素之一。本论文提出了一种基于改进遗传算法的圆阵列方向图联合优化方法。该方法综合考虑圆阵列天线元素的位置和幅度权值,以最小化方向图的主瓣宽度和副瓣深度为目标,同时考虑到各个天线元素之间的互相干扰。通过实验验证,该方法在优化方向图的同时能够有效降低互相干扰,提高系统的通信质量和覆盖范围。关键词:圆阵列、方向图、遗
基于停滞检测粒子群算法的阵列天线方向图综合.docx
基于停滞检测粒子群算法的阵列天线方向图综合AbstractInthispaper,anovelapproachofusingthestagnationdetectionparticleswarmoptimizationalgorithmforthesynthesisofantennaarraydirectionaldiagramisproposed.Theproposedmethodaimstooptimizethearrayfactoroftheantennawhiletakingintoaccount