
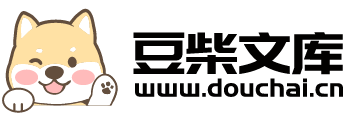
基于MED的滚动轴承故障特征提取方法及其应用.docx
快乐****蜜蜂
在线预览结束,喜欢就下载吧,查找使用更方便
相关资料
基于MED的滚动轴承故障特征提取方法及其应用.docx
基于MED的滚动轴承故障特征提取方法及其应用摘要:随着工业生产的不断发展以及机械设备的广泛应用,滚动轴承作为重要的机械部件之一,在现代工业中扮演着重要的角色。而滚动轴承的故障对于机械设备的稳定运行和生产效率有着重要的影响。因此,基于MED的滚动轴承故障特征提取方法的研究和应用,具有重要的理论和实践意义。本文通过文献调研,着重介绍MED(多尺度熵)在滚动轴承故障特征提取中的应用,分别从理论原理和实验研究两方面进行阐述。针对传统故障诊断方法存在的局限性,基于MED的特征提取方法能够快速准确地诊断滚动轴承的故障
基于MED及FSK的滚动轴承微弱故障特征提取.docx
基于MED及FSK的滚动轴承微弱故障特征提取摘要本文基于MED(最小二乘能量差)和FSK(频率偏移键控)方法,研究了滚动轴承微弱故障的特征提取。首先通过模拟产生不同程度的滚动轴承故障信号,并对其进行了预处理。然后使用MED方法对信号进行特征提取和分类,并通过绘制MED谱图的方法对滚动轴承故障进行了模式识别。接着,使用FSK方法提取滚动轴承振动信号的频率特征,并进行视觉化展示。实验结果表明,本文提出的方法能够有效地识别滚动轴承的微弱故障特征,对于滚动轴承的健康状态监测具有重要的应用价值。关键词:滚动轴承、微
MED方法及在滚动轴承故障诊断中的应用.docx
MED方法及在滚动轴承故障诊断中的应用介绍MED方法,又叫MachineryFaultDiagnosisExpertSystem,是一种机械故障诊断专家系统。该系统可以根据多参数数据对机械设备的运行状态进行判断,从而判断哪些部件存在故障,以及故障的具体类型。在滚动轴承故障诊断中,MED方法也得到了广泛的应用。利用该方法,可以快速准确地诊断轴承的故障类型,及时实施维修。MED方法基本原理MED方法基于以下两个基本原理:1.多参数分析方法我们知道,一台机械设备的工作过程中,不止一个参数会产生变化,例如温度、震
基于FDM和MED的滚动轴承故障诊断方法.pptx
基于FDM和MED的滚动轴承故障诊断方法目录添加章节标题滚动轴承故障诊断方法概述滚动轴承故障诊断的意义常见的滚动轴承故障诊断方法基于FDM的滚动轴承故障诊断方法FDM的基本原理FDM在滚动轴承故障诊断中的应用FDM的优势与局限性基于MED的滚动轴承故障诊断方法MED的基本原理MED在滚动轴承故障诊断中的应用MED的优势与局限性基于FDM和MED的滚动轴承故障诊断方法比较FDM和MED的优缺点比较FDM和MED的适用范围比较FDM和MED的互补性分析基于FDM和MED的滚动轴承故障诊断方法应用案例应用案例一
基于MED和CEEMD的滚动轴承故障诊断方法研究.docx
基于MED和CEEMD的滚动轴承故障诊断方法研究基于MED和CEEMD的滚动轴承故障诊断方法研究摘要:滚动轴承是旋转机械中常见的关键部件,其运行状态的监测和故障诊断对于机械设备的可靠性和安全性至关重要。本文提出一种基于最大熵差和集合经验模态分解的滚动轴承故障诊断方法(MED-CEEMD)。该方法首先利用最大熵差对轴承振动信号进行预处理,然后应用集合经验模态分解对预处理后的信号进行分解,并提取有用的特征参数。最后,利用支持向量机对特征参数进行分类和诊断。实验结果表明,该方法在滚动轴承故障的诊断方面具有较好的