
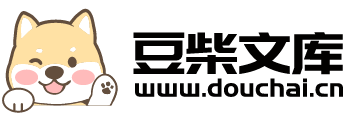
改进的遗传算法优化BP神经网络.docx
快乐****蜜蜂
在线预览结束,喜欢就下载吧,查找使用更方便
相关资料
改进的遗传算法优化BP神经网络.docx
改进的遗传算法优化BP神经网络IntroductionThebackpropagation(BP)neuralnetworkhasbeenwidelyusedinvariousfieldsduetoitspowerfulnonlinearmappingandlearningability.However,itisachallengingtasktodeterminetheoptimalparametersofBPneuralnetworkforaspecifictask.Traditionaloptimi
改进遗传算法优化的BP神经网络高炉煤气预测.docx
改进遗传算法优化的BP神经网络高炉煤气预测Title:OptimizationofBPNeuralNetworkforPredictingBlastFurnaceGasusingGeneticAlgorithmIntroduction:Theoptimizationofforecastingmodelsplaysacrucialroleinvariousindustries,includingthesteelindustry.Blastfurnacegaspredictionisanessentialco
基于改进的自适应遗传算法优化BP神经网络.docx
基于改进的自适应遗传算法优化BP神经网络改进的自适应遗传算法优化BP神经网络摘要:近年来,人工神经网络在实际应用中得到了广泛的应用。然而,BP神经网络在处理复杂问题时存在着训练时间长、易陷入局部最优等问题。针对这些问题,本文提出了一种基于改进的自适应遗传算法优化BP神经网络的方法。通过引入自适应遗传算法来优化BP神经网络的权重和阈值,以提高网络的训练速度和准确性。实验结果表明,该方法在处理复杂问题时具有较好的性能和稳定性。关键词:BP神经网络;自适应遗传算法;权重和阈值优化;训练速度和准确性1.引言自适应
基于改进的遗传算法优化BP神经网络的车险欺诈识别模型.pptx
基于改进的遗传算法优化BP神经网络的车险欺诈识别模型目录遗传算法优化BP神经网络的基本原理遗传算法概述BP神经网络的基本原理遗传算法优化BP神经网络的基本步骤改进的遗传算法优化BP神经网络的方法改进的遗传算法介绍改进的遗传算法优化BP神经网络的具体步骤改进的遗传算法优化BP神经网络的优势车险欺诈识别的应用场景和需求车险欺诈识别的背景和意义车险欺诈识别的应用场景和需求分析车险欺诈识别的技术挑战和难点基于改进的遗传算法优化BP神经网络的车险欺诈识别模型构建数据预处理和特征提取模型训练和参数调整模型评估和性能测
基于改进的遗传算法优化BP神经网络的车险欺诈识别模型.docx
基于改进的遗传算法优化BP神经网络的车险欺诈识别模型基于改进的遗传算法优化BP神经网络的车险欺诈识别模型摘要:车险欺诈是保险行业中普遍存在的问题,对其进行及时准确的识别和预测对于保险公司来说至关重要。本文基于改进的遗传算法优化BP神经网络,提出了一种车险欺诈识别模型。首先,通过改进遗传算法选择合适的神经网络结构和初始化参数。其次,利用遗传算法进行进化权值和阈值的优化。最后,利用BP神经网络进行车险欺诈的分类学习和预测。通过实验验证,本文提出的模型在车险欺诈识别中具有良好的性能和准确度。关键词:车险欺诈、遗