
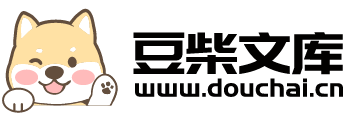
基于RBF神经网络的混凝土泵车臂架运动学逆解.docx
骑着****猪猪
在线预览结束,喜欢就下载吧,查找使用更方便
相关资料
基于RBF神经网络的混凝土泵车臂架运动学逆解.docx
基于RBF神经网络的混凝土泵车臂架运动学逆解为题目,写不少于1200的论文1.IntroductionNowadays,theconstructionindustryisrapidlydevelopingworldwide,andtheconcretepumptruckisconsideredasignificantdeviceintheconstructionsite.Itiscrucialequipmentthathelpstoplaceready-mixconcreteatdifferentelev
基于RBF神经网络间接求取运动学逆解的研究.docx
基于RBF神经网络间接求取运动学逆解的研究标题:基于RBF神经网络的间接运动学逆解研究摘要:运动学逆解在机器人控制领域具有重要的理论和应用价值。本文针对复杂机器人系统的运动学逆解问题,提出一种基于RBF神经网络的间接运动学逆解方法。该方法通过对机器人系统的正向运动学进行建模,将运动学逆问题转化为非线性函数拟合问题,然后利用RBF神经网络进行非线性函数的逼近和求解。经过实验验证,该方法具有较高的准确性和实时性,为机器人系统在不同工作空间中的运动控制提供了一种有效的解决方案。1.引言运动学逆解是机器人控制中的
基于改进门控循环神经网络的机械臂逆运动学解.docx
基于改进门控循环神经网络的机械臂逆运动学解一、研究背景和意义随着科技的不断发展,机械臂在工业生产、医疗护理、家庭服务等领域的应用越来越广泛。逆运动学(InverseKinematics)是机械臂控制中的一个重要问题,它研究如何根据末端执行器的位置和姿态来确定机械臂的运动轨迹。传统的逆运动学方法主要依赖于解析解或数值求解,这些方法在一定程度上可以解决实际问题,但计算复杂度较高,且对初始条件和模型参数较为敏感。研究一种高效、准确的逆运动学方法具有重要的理论和实际意义。深度学习技术在图像识别、语音识别等领域取得
基于RBF神经网络的机械手逆运动学求解.docx
基于RBF神经网络的机械手逆运动学求解摘要逆运动学问题一直是机器人技术领域的研究热点之一。本文针对机械手的逆运动学问题,提出基于径向基函数(RadialBasisFunction,简称RBF)神经网络的求解方法。该方法利用神经网络的高精度和快速学习能力,对机器人运动学模型进行建模,在实际运动控制中实现了高精度的轨迹跟踪。实验结果表明,该方法在机械手逆运动学问题求解中具有较高的精度和计算速度,是一种有效的机器人控制方法。关键词:机械手,逆运动学,径向基函数神经网络,轨迹跟踪,控制1.引言逆运动学问题是机器人
基于BP神经网络的救援机械臂的逆运动学求解.docx
基于BP神经网络的救援机械臂的逆运动学求解基于BP神经网络的救援机械臂逆运动学求解摘要:救援机械臂是一种重要的救援设备,它在各种复杂环境下执行任务,为人们提供生命安全保障。逆运动学是救援机械臂运动控制中的关键问题之一,其主要任务是根据目标位置确定各关节的角度,从而实现所需的动作。为了解决逆运动学求解问题,本文提出了一种基于BP神经网络的方法,并对其进行了模拟实验与性能分析。实验结果表明,基于BP神经网络的逆运动学求解方法具有较高的精确度和鲁棒性,能够有效应对各种复杂情况下的求解需求。1.引言救援机械臂是应