
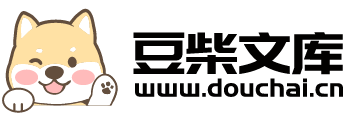
基于基因表达数据的肿瘤分类方法研究的中期报告.docx
快乐****蜜蜂
在线预览结束,喜欢就下载吧,查找使用更方便
相关资料
基于基因表达数据的肿瘤分类方法研究的中期报告.docx
基于基因表达数据的肿瘤分类方法研究的中期报告摘要:肿瘤是一类常见的恶性疾病,肿瘤分类对于治疗和预后的预测具有重要意义。近年来,基因表达数据在肿瘤学中的应用日益广泛,本研究旨在探究基于基因表达数据的肿瘤分类方法,以提高对肿瘤治疗和预后的准确预测。本研究采用公开的基因表达数据集,利用机器学习方法对不同类型的肿瘤进行分类。首先,对数据进行预处理,包括数据清洗和归一化。然后,使用主成分分析(PCA)对数据进行特征选择和降维。接着,分别使用支持向量机(SVM)、随机森林(RF)和神经网络(NN)三种机器学习方法对数
基于基因表达数据的肿瘤亚型多类别分类与分析的中期报告.docx
基于基因表达数据的肿瘤亚型多类别分类与分析的中期报告一、研究背景癌症是目前世界范围内的一大重要疾病。肿瘤的发生和发展与细胞的基因表达密切相关。利用基因芯片等技术能够获取大量的基因表达数据,为肿瘤的诊断和分类提供了新的手段。近年来,将机器学习算法应用于肿瘤亚型多类别分类和分析领域取得了显著的进展。二、研究目的本研究旨在基于基因表达数据进行肿瘤亚型多类别分类和分析,并探究其在临床治疗中的应用前景。三、研究方法1.数据预处理从公共数据库中下载多个肿瘤基因表达数据集,并进行质量控制和预处理,包括数据清洗、归一化、
基于基因表达数据的样本分类研究的中期报告.docx
基于基因表达数据的样本分类研究的中期报告尊敬的评委老师,大家好!我做的研究是基于基因表达数据的样本分类研究,现在我来给大家做一下中期报告。研究背景和目的基因表达是指细胞中基因转录为mRNA的过程,通过分析这些mRNA的表达水平可以了解不同组织、不同疾病的生物学特征。在现代生物学研究中,基因表达数据成为了非常重要的数据类型。本研究的目的是通过对基因表达数据的分析,建立分类模型,对不同样本进行分类,从而为疾病的诊断和治疗提供参考。研究方法和进展首先,我们获得了基因表达数据,并对数据进行了预处理,包括数据清洗、
基于基因表达谱的数据挖掘方法研究的中期报告.docx
基于基因表达谱的数据挖掘方法研究的中期报告尊敬的评委:我是XX,我今天来介绍一下我的中期报告,我的研究是基于基因表达谱的数据挖掘方法研究。首先,我简要介绍了基因表达谱以及数据挖掘的概念和意义,接着介绍了一些常用的基因表达谱数据处理方法和数据挖掘方法,包括聚类分析、主成分分析、因子分析、支持向量机等,还介绍了一些相关领域的研究进展和应用实例。然后,我提出了我自己的研究思路和方法,该研究主要针对基因表达谱数据的非线性特征和高维问题,在改进传统的数据挖掘方法的基础上,结合深度学习技术,提出了一种基于卷积神经网络
基于邻接谱分解的基因表达谱数据分类研究的中期报告.docx
基于邻接谱分解的基因表达谱数据分类研究的中期报告1.介绍基因表达谱数据分类是生物信息学领域的重要问题之一。邻接谱分解是一种新的机器学习方法,能够有效地解决图像识别、自然语言处理等领域中的分类问题。本研究旨在探索邻接谱分解方法在基因表达谱数据分类中的应用。2.数据集本研究使用了公开的基因表达谱数据集GSE2034,该数据集包含了1091个样本,每个样本包含22283个基因表达量数据。这些样本分为两类,即乳腺癌组织和正常组织。3.方法本研究采用邻接谱分解方法对基因表达谱数据进行分类。具体步骤如下:(1)构建邻