
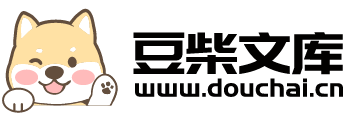
统计参数在变压器局部放电模式识别中的应用.pdf
as****16
在线预览结束,喜欢就下载吧,查找使用更方便
相关资料
统计参数在变压器局部放电模式识别中的应用.pdf
第卷第期352高电压技术Vol.35No.22009年2月HighVoltageEngineeringFeb.2009·277·统计参数在变压器局部放电模式识别中的应用胡文堂1,高胜友2,余绍峰1,谈克雄2,高文胜2(1.浙江省电力试验研究院,杭州310014;2.清华大学电机系电力系统及发电设备控制和仿真国家重点实验室,北京100084)摘要:对局部放电进行模式识别可以了解放电类型和严重程度,为故障诊断和检修提供参考依据。为此将Weibull统计分布参数用于局部放电模式识别当中,采用仿真分析和模型试验的
统计参数用于局部放电模式识别的研究.pdf
4Aug.2002~IG~VOLTAGEENGINEERINGVol.28No.8统计参数用于局部放电模式识别的研究tatisticalParameterMethodforPDPatternRecognition唐炬l王静l李剑l谭志红2(l.重庆大学高电压与新技术教育部重点实验室重庆400044;2.西昌电业局四川6l5000)摘要通过研究局部放电二维谱图的统计特性对局部放用针板电极针尖曲率半径<0.lmm,油中尖板放电脉冲幅值谱图进行WeiBull分析获得尺度参数o和形状电模型见图空气中沿面放电模型见
神经网络在变压器超高频局部放电模式识别中的应用.pdf
万方数据放电模式识别中的应用神经网络在变压器超高频局部殷录民1,张慧芬2引言transformersramm舯ent,ShandongApplicationofneuralnetworkinUHFPDpatternrecognition250022,China)Algorithm(AGA)withBack—Propagation(BP)algorithm,anwords:uhra—high—frequencynetwork(NN)0神经网络用于局部放电模式识别提高了识别的主要有BP算法和AGA算法.BP算
变压器局部放电的分步特征分析与模式识别.docx
变压器局部放电的分步特征分析与模式识别Title:PartialDischargeAnalysisandPatternRecognitioninTransformersAbstract:Partialdischarge(PD)isaphenomenonthatoccursinelectricalinsulationsystemsandistheleadingcauseofsystemfailuresinpowertransformers.Therefore,itiscrucialtodevelopeffe
基于深度学习的变压器局部放电模式识别研究.pptx
基于深度学习的变压器局部放电模式识别研究目录添加章节标题变压器局部放电模式识别的背景和意义变压器局部放电的危害模式识别在变压器局部放电检测中的重要性深度学习在模式识别领域的应用及优势变压器局部放电模式识别的传统方法传统方法的局限性和挑战传统方法在变压器局部放电模式识别中的应用案例传统方法的效果评估和比较基于深度学习的变压器局部放电模式识别方法深度学习模型的选择和设计数据预处理和特征提取方法模型训练和优化过程模型在变压器局部放电模式识别中的应用效果和优势实验设计和结果分析数据集的收集和处理实验方案设计和实施