
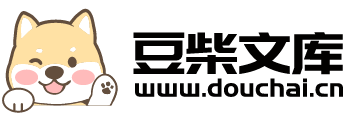
I-MINER环境下聚类分析算法研究与实现-聚类算法DBSCAN和FCM算法实现.doc
努力****恨风
亲,该文档总共53页,到这已经超出免费预览范围,如果喜欢就直接下载吧~
相关资料
I-MINER环境下聚类分析算法研究与实现-聚类算法DBSCAN和FCM算法实现.doc
西南交通大学本科毕业设计(论文)第PAGEVI页I-MINER环境下聚类分析算法研究与实现摘要由于计算机和数据采集技术的进步,数据挖掘获得了非常广泛的应用。数据挖掘帮助用户发现隐藏在大型数据库种的规律和模式,它融合了人工智能、统计、机器学习、模式识别和数据库等多种学科的理论、方法与技术。数据挖掘模型包括决策树、关联规则、聚类、神经网络、粗糙集、概念格、遗传算法、序列模式、贝叶斯、支持向量机、模糊集和基于案例的推理。其中,聚类是数据挖掘领域的核心技术,被广泛应用于相似搜索、顾客划分、趋势分析、金融投资
I-MINER环境下聚类分析算法研究与实现-聚类算法DBSCAN和FCM算法实现.doc
西南交通大学本科毕业设计(论文)第PAGEVI页I-MINER环境下聚类分析算法研究与实现摘要由于计算机和数据采集技术的进步,数据挖掘获得了非常广泛的应用。数据挖掘帮助用户发现隐藏在大型数据库种的规律和模式,它融合了人工智能、统计、机器学习、模式识别和数据库等多种学科的理论、方法与技术。数据挖掘模型包括决策树、关联规则、聚类、神经网络、粗糙集、概念格、遗传算法、序列模式、贝叶斯、支持向量机、模糊集和基于案例的推理。其中,聚类是数据挖掘领域的核心技术,被广泛应用于相似搜索、顾客划分、趋势分析、金融投资
I-MINER环境下聚类分析算法研究与实现-聚类算法DBSCAN和FCM算法实现.doc
西南交通大学本科毕业设计(论文)第VI页I-MINER环境下聚类分析算法研究与实现摘要由于计算机和数据采集技术的进步,数据挖掘获得了非常广泛的应用。数据挖掘帮助用户发现隐藏在大型数据库种的规律和模式,它融合了人工智能、统计、机器学习、模式识别和数据库等多种学科的理论、方法与技术。数据挖掘模型包括决策树、关联规则、聚类、神经网络、粗糙集、概念格、遗传算法、序列模式、贝叶斯、支持向量机、模糊集和基于案例的推理。其中,聚类是数据挖掘领域的核心技术,被广泛应用于相似搜索、顾客划分、趋势分析、金融投资和信息检索等领
基于粒子群算法的DBSCAN聚类算法研究.docx
基于粒子群算法的DBSCAN聚类算法研究基于粒子群算法的DBSCAN聚类算法研究摘要:随着大数据时代的到来,聚类算法成为了处理海量数据的重要工具。DBSCAN是一种经典的基于密度的聚类算法,但在处理大规模数据集时存在着效率较低的问题。粒子群算法(PSO)是一种优化算法,具有全局搜索优势,在聚类问题中也取得了良好的应用效果。本文研究将粒子群算法与DBSCAN相结合,以提高聚类算法的效率与准确性。1.引言聚类是一种常见的数据挖掘技术,它通过将相似的数据样本划分到同一类别中,发现数据之间的内在关系和结构。DBS
DBSCAN聚类算法.pptx
DBSCAN聚类算法目录基于密度聚类算法基于密度聚类算法密度的定义DBSCAN点分类DBSCAN点分类DBSCAN:核心点、边界点和噪音点DBSCAN:核心点、边界点和噪音点DBSCAN算法概念DBSCAN算法概念DBSCAN算法概念示例解答DBSCAN算法原理DBSCAN算法伪代码DBSCAN运行效果好的时候DBSCAN运行不好的效果DBSCAN算法的一些问题DBSCAN算法的一些问题如何合适选取EPS和MinPtsDBSCAN算法的优缺点DBSCAN的应用DBSCAN的应用DBSCAN的应用DBSCA