
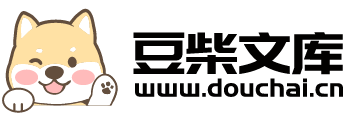
基于多核学习支持向量机的音乐流派分类.docx
映雁****魔王
亲,该文档总共13页,到这已经超出免费预览范围,如果喜欢就直接下载吧~
相关资料
基于多核学习支持向量机的音乐流派分类.docx
基于多核学习支持向量机的音乐流派分类摘要:针对不同特征向量下选择最优核函数的学习方法问题将多核学习支持向量机(MKSVM)应用于音乐流派自动分类中提出了将最优核函数进行加权组合构成合成核函数进行流派分类的方法。多核分类学习能够针对不同的声学特征采用不同的最优核函数并通过学习得到各个核函数在分类中的权重从而明确各声学特征在流派分类中的权重为音乐流派分类中特征向量的分析和选择提供了一个清晰、明确的结果。在ISMIR2011竞赛数据集上验证了提出的基于多核学习支持向量机(M
基于多核学习支持向量机的音乐流派分类.docx
基于多核学习支持向量机的音乐流派分类摘要:针对不同特征向量下选择最优核函数的学习方法问题将多核学习支持向量机(MKSVM)应用于音乐流派自动分类中提出了将最优核函数进行加权组合构成合成核函数进行流派分类的方法。多核分类学习能够针对不同的声学特征采用不同的最优核函数并通过学习得到各个核函数在分类中的权重从而明确各声学特征在流派分类中的权重为音乐流派分类中特征向量的分析和选择提供了一个清晰、明确的结果。在ISMIR2011竞赛数据集上验证了提出的基于多核学习支持向量机(M
基于支持向量机的音乐自动分类.docx
基于支持向量机的音乐自动分类基于支持向量机的音乐自动分类摘要:音乐自动分类是一种非常有挑战性且具有广泛应用的任务。本论文提出了一种基于支持向量机(SupportVectorMachine,SVM)的音乐自动分类方法。通过对音频信号进行特征提取,并将特征向量作为SVM的输入,可以实现对音乐进行自动分类。实验结果表明,该方法在音乐分类准确度和效率方面具有明显优势。1.引言随着数字音乐时代的到来,音乐库中的音乐数量快速增加。为了方便用户浏览和搜索音乐,音乐自动分类成为了一个非常重要的问题。音乐自动分类是一种将音
一种基于特征加权模糊支持向量机的音乐流派分类方法及系统.pdf
本发明涉及一种基于特征加权模糊支持向量机的音乐流派分类方法及系统,属于音乐内容检索及模式识别技术领域。本发明的分类器选择模糊支持向量机,能够根据不同输入样本对分类贡献的不同,赋以相应隶属度,目的在于能够很好的减少噪声的影响;用reliefF特征选择算法计算出的各特征权重,用于对模糊支持向量机的隶属度的确定方法,考虑到了不同权重的特征对分类影响大小的不同;针对盲区不可分点,用该点到各个类中心的加权欧氏距离进行多类别概率划分,符合当下音乐多元化的实质。
基于多核支持向量机的网络流量分类方法研究.pptx
汇报人:CONTENTSPARTONEPARTTWO网络流量分类的必要性现有的网络流量分类方法及其局限性研究目的与意义PARTTHREE多核支持向量机算法概述网络流量分类技术发展历程相关研究工作综述PARTFOUR基于多核支持向量机的网络流量分类算法设计算法实现与实验验证实验结果分析与比较PARTFIVE实验数据集与预处理实验结果展示与对比分析结果讨论与解释PARTSIX研究成果总结创新点与贡献未来研究方向与展望汇报人: