
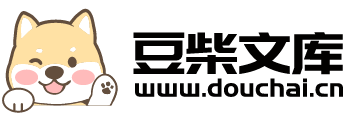
一种LDA与SVM混合的多类分类方法.docx
快乐****蜜蜂
在线预览结束,喜欢就下载吧,查找使用更方便
相关资料
一种LDA与SVM混合的多类分类方法.docx
一种LDA与SVM混合的多类分类方法IntroductionTheclassificationoftextdocumentshasbeenawidelyresearchedtopicinthefieldofmachinelearning.Inrecentyears,therehasbeenanincreasinginterestintheuseoftopicmodels,suchasLatentDirichletAllocation(LDA),fordocumentclassification.LDAis
一种LDA和聚类融合的SVM多类分类方法.docx
一种LDA和聚类融合的SVM多类分类方法一种结合LDA和聚类的SVM多类分类方法摘要:在机器学习领域中,多类分类问题一直是一个重要的研究方向。本论文提出了一种结合LatentDirichletAllocation(LDA)和聚类的支持向量机(SVM)多类分类方法。通过将文本数据转化为主题分布向量的形式,使用LDA模型对文本进行主题建模,得到文档的主题分布特征。然后,使用聚类算法对文档进行聚类,将相似的文档归为同一类。最后,使用SVM对每个类别进行二分类,得到最终的多类分类结果。通过实验验证,我们的方法在多
融合LDA的多类SVM方法研究.docx
融合LDA的多类SVM方法研究随着信息技术的不断发展和人类社会对数据处理能力的迫切需求,机器学习技术逐渐应用于各个领域,成为重要的研究方向之一。其中,支持向量机是一种常用的分类算法,但是在多类问题上的表现并不理想。针对这一问题,本文介绍一种融合LDA的多类SVM方法,可以更好地适用于多类问题。支持向量机最初被提出是在二分类问题中处理线性不可分数据集的方法。随着研究的深入,支持向量机被推广至非线性情况,并在多种领域得到应用。然而,在多类问题上,传统的支持向量机表现并不如人意。主要原因是支持向量机只能解决二分
LDA特征扩展的多类SVM短文本分类方法研究.docx
LDA特征扩展的多类SVM短文本分类方法研究LDA特征扩展的多类SVM短文本分类方法研究摘要:本文提出了一种LDA特征扩展的多类支持向量机(SVM)短文本分类方法。传统的短文本分类方法在处理维度较高的文本特征时存在一定的局限性,因此引入了主题模型LDA(LatentDirichletAllocation)对文本进行特征降维和扩展。在LDA特征扩展的基础上,采用多类支持向量机进行短文本分类。实验结果表明,该方法在短文本分类任务中具有较好的性能。关键词:LDA;支持向量机;短文本分类;特征扩展1.引言随着互联
基于特征距离的多类SVM分类方法研究.docx
基于特征距离的多类SVM分类方法研究基于特征距离的多类SVM分类方法研究摘要:支持向量机(SupportVectorMachine,SVM)是一种常用的机器学习算法,在二分类问题中表现出色。然而,在多类别分类问题中,传统的SVM模型仍然存在一些挑战,比如样本不均衡、特征维度高等。为了解决这些问题,本文研究了基于特征距离的多类SVM分类方法,并对其进行了详细的分析和评估。1.引言支持向量机是一种通过寻找最优超平面来解决分类问题的算法。在二分类问题中,SVM通过找到一个划分超平面,使得同一类别的样本尽可能靠近