
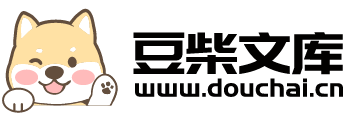
基于局部PLS的多输出过程自适应软测量建模方法(英文).docx
快乐****蜜蜂
在线预览结束,喜欢就下载吧,查找使用更方便
相关资料
基于局部PLS的多输出过程自适应软测量建模方法(英文).docx
基于局部PLS的多输出过程自适应软测量建模方法(英文)BasicIntroductiontotheTopic:Theconceptofsoftsensingorsoftmeasurementiswidelyusedintheprocessindustry.Itisanon-invasivemethodofmeasuringvariousprocessvariableswhicharedifficulttomeasurewithconventionalsensors.Softsensingisachieve
时序局部加权自适应核PLS软测量建模及其应用.docx
时序局部加权自适应核PLS软测量建模及其应用时序局部加权自适应核PLS软测量建模及其应用摘要:随着工业过程的发展和复杂性的增加,软测量成为工业过程监测和控制中不可或缺的手段。传统的软测量方法往往假设过程数据服从线性模型,忽略了数据的非线性特性。为了更准确地描述工业过程的非线性行为,本文提出了一种时序局部加权自适应核PLS(PartialLeastSquare)软测量建模方法,并将其应用于工业过程中。实验结果证明了该方法的有效性和可行性。关键词:软测量、时序、局部加权、自适应核PLS、建模、应用1.引言随着
基于PLS和LSSVM的两阶段软测量建模方法.docx
基于PLS和LSSVM的两阶段软测量建模方法摘要:随着现代制造企业在生产过程中的追求高效、高质量和可持续发展,越来越多的制造企业开始应用软测量技术以确保生产过程的良好控制。本文针对软测量中的建模问题,提出了一种基于PLS和LSSVM的两阶段软测量建模方法。该方法以PLS为第一阶段建模,然后将建模结果用于LSSVM建模,以更准确地描述生产过程中的关键参数。通过对三个实际工业案例的仿真实验,验证了该方法的有效性。关键词:软测量;PLS;LSSVM;建模1.引言随着制造业的快速发展,如何提高生产过程的质量和效率
集成自适应核PLS软测量建模方法及应用研究的开题报告.docx
集成自适应核PLS软测量建模方法及应用研究的开题报告开题报告题目:集成自适应核PLS软测量建模方法及应用研究一、选题背景软测量建模是利用数学模型对生产过程中的关键变量进行预测与控制,从而提高生产效率和质量稳定性的一种重要方法。在工业生产中,利用软测量建模可以对关键产品参数进行实时监控和预测,从而避免对过程的不必要干预和降低由于测量误差、传感器故障等因素导致的生产不稳定性。目前,已有很多软测量建模方法,其中PLS(PartialLeastSquares)是一种常见的建模方法,它可以有效地处理高维数据和变量之
集成自适应核PLS软测量建模方法及应用研究的任务书.docx
集成自适应核PLS软测量建模方法及应用研究的任务书任务书一、任务背景随着工业生产向自动化、信息化、智能化方向发展,工业过程监测与控制变得越来越重要。软测量建模作为一种基于数据分析的方法,可以有效地监测和控制工业过程中的关键参数,提高生产效率和产品质量。其中,基于核主元分析的PLS(PartialLeastSquares)方法因其能够在高维数据空间中找到最佳的线性关系而受到广泛关注。然而,对于非线性的过程和多变量之间的复杂关系建模,传统的核PLS方法可能存在一定的局限性。自适应核PLS方法通过引入核函数,并