
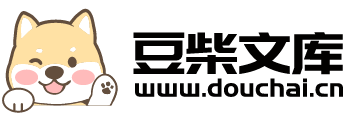
基于灰色神经网络的油料储备预测模型研究.docx
快乐****蜜蜂
在线预览结束,喜欢就下载吧,查找使用更方便
相关资料
基于灰色神经网络的油料储备预测模型研究.docx
基于灰色神经网络的油料储备预测模型研究IntroductionThepredictionofoilreserveisanimportantaspectofenergymanagement.Basedonaccurateprediction,governmentandorganizationscanplanandexecutetheirpoliciesandstrategiesrelatedtooilmanagement,production,andexploration.Thepredictionofoi
基于灰色神经网络的预测模型研究.docx
基于灰色神经网络的预测模型研究随着人们对于预测技术需求的不断增长,各类预测模型的研究也越来越受到广泛关注。其中,基于灰色神经网络的预测模型是当前研究的热点之一。本文将就该模型进行详细的介绍与分析。一、灰色神经网络的概述灰色神经网络(GreyNeuralNetwork,简称GNN)是一种以灰色模型为依据的神经网络。灰色模型是一种非常有效的短期预测方法,由于其对于样本数据的灵活性,一般被用于小样本、非线性、不确定的系统进行短期预测分析。灰模型有两种基本形式:GM(1,1)和GM(2,1)。其中,GM(1,1)
基于多维灰色模型与神经网络的销售预测模型研究.docx
基于多维灰色模型与神经网络的销售预测模型研究基于多维灰色模型与神经网络的销售预测模型研究摘要:随着市场竞争激烈程度的不断加剧,对于企业而言,如何准确预测销售额成为了一项关键任务。本文针对销售预测问题,提出了一种基于多维灰色模型与神经网络相结合的销售预测模型。首先,利用多维灰色模型对销售数据进行初步建模与预测,然后将多维灰色模型的输出结果作为神经网络的输入,进一步优化模型的预测精度。实验证明,这种基于多维灰色模型与神经网络的销售预测模型具有较高的准确性和可靠性。关键词:多维灰色模型,神经网络,销售预测,准确
基于灰色神经网络的预测模型研究的开题报告.docx
基于灰色神经网络的预测模型研究的开题报告一、研究背景及意义经济预测作为一种预测未来的方法,对于政府制定相关政策、企业决策以及投资者的投资策略有着至关重要的作用。传统的经济预测方法主要包括时间序列分析、回归分析等,但是这些方法往往需要大量的数据,预测结果具有一定的滞后性,且存在较大误差。在新时代背景下,采用新型的经济预测方法具有更高的意义。灰色系统理论是近年来发展较快并在多个领域得到广泛应用的一种预测方法。灰色神经网络是将灰色系统理论与神经网络理论相结合的模型,具有模型简单、预测精度高等优点。因此,基于灰色
基于灰色神经网络模型的基坑变形预测研究.docx
基于灰色神经网络模型的基坑变形预测研究基于灰色神经网络模型的基坑变形预测研究摘要:基坑工程的变形预测一直是建筑工程领域的一个重要研究方向。近年来,灰色神经网络模型(GreyNeuralNetwork,GNN)的应用逐渐得到了广泛关注。本文针对基坑变形预测的问题,通过建立灰色神经网络模型,进行了相关研究。研究结果表明,灰色神经网络模型能够有效地预测基坑变形,并具有较高的精确度和可行性。关键词:基坑工程;变形预测;灰色神经网络模型;精确度;可行性1.引言基坑工程的变形预测对于工程安全和成本控制具有重要意义。目