
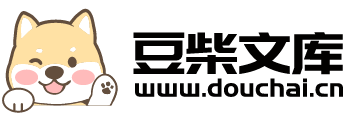
基于改进高斯混合模型的运动物体的图像检测.docx
快乐****蜜蜂
在线预览结束,喜欢就下载吧,查找使用更方便
相关资料
基于改进高斯混合模型的运动物体的图像检测.docx
基于改进高斯混合模型的运动物体的图像检测摘要:本文提出了一种改进高斯混合模型(GMM)的运动物体图像检测方法。传统的GMM方法在处理目标跟踪时容易受到光照条件、背景复杂度等因素的影响,造成误检和漏检现象。本文通过增加背景图像的反比权值、引入颜色模型等方法,对GMM进行改进,增强了对运动物体的检测效果。实验结果表明,该方法在复杂背景下有较强的适应性和鲁棒性,能够有效地检测出运动物体。关键词:运动物体检测;高斯混合模型;背景建模Abstract:Inthispaper,animprovedGaussianMi
基于改进的混合高斯背景模型的运动目标检测.docx
基于改进的混合高斯背景模型的运动目标检测混合高斯背景模型是一种常用的目标检测方法,它通过建立当前画面和背景的概率模型来判断画面中的目标是否存在。然而,在实际场景中,场景不断变化,诸如光照变化、瞬间运动、背景场景切换等影响因素都会对检测效果造成一定的影响。基于此,本文提出一种改进的混合高斯背景模型算法,旨在提高运动目标检测的稳定性和准确性。一、基本原理混合高斯背景模型是通过建立当前画面的高斯背景模型来判断目标是否存在的一种算法。在该模型中,每个像素点都是由多个高斯分布组成。对于一个像素点,它的高斯分布包括背
基于改进混合高斯模型的运动目标检测研究.docx
基于改进混合高斯模型的运动目标检测研究基于改进混合高斯模型的运动目标检测研究摘要:在计算机视觉领域,运动目标检测是一个关键的研究领域,它在许多应用中具有重要的实际意义。本文提出了一种改进的混合高斯模型,用于有效地进行运动目标检测。传统的混合高斯模型在处理快速运动目标时存在定位不准确、运动模糊等问题。针对这些问题,本文通过引入运动信息和自适应更新机制,对传统的混合高斯模型进行了改进。实验证明,改进后的混合高斯模型在运动目标检测方面具有更好的性能,能够更准确地定位目标并消除运动模糊。关键词:混合高斯模型,运动
基于高斯混合模型联合CamShift的运动图像检测跟踪方法.docx
基于高斯混合模型联合CamShift的运动图像检测跟踪方法一、引言在模式识别、计算机视觉和机器学习领域中,目标检测以及跟踪一直是热门的话题。目标检测是指识别图像或视频中的目标对象,并确定其在图像中的位置。而目标跟踪则是识别目标目前的位置并在连续的帧中追踪它的位置。为了实现高效的目标检测与跟踪,本文提出了一个基于高斯混合模型(GMM)联合CamShift的运动图像检测跟踪方法。二、GMM模型GMM是一种混合模型,它将一个复杂的分布表示为许多单一的高斯分布的混合。GMM是目标检测与跟踪中常用的方法,因为它可以
基于改进混合高斯模型的运动目标检测研究的开题报告.docx
基于改进混合高斯模型的运动目标检测研究的开题报告一、选题背景:在自动驾驶、视频监控等领域,运动目标的检测是最基本的任务之一。传统的运动目标检测算法主要采用基于光流法、背景差分等方法。然而,这些方法基本上依赖于静态摄像头,对于动态摄像头的场景来说,效果并不理想。因此,深度学习引入到运动目标检测领域受到了广泛关注。其中,混合高斯模型也是一种常见的背景建模方法,可以对图像背景进行动态推定,进而提高运动目标检测的准确度。但是,传统的混合高斯模型受到光照变化等因素的影响较大,进而导致漏检和误检等问题。因此,如何改进