
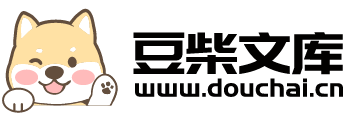
基于Matlab和BP神经网络的爆破振动预测系统.docx
骑着****猪猪
在线预览结束,喜欢就下载吧,查找使用更方便
相关资料
基于Matlab和BP神经网络的爆破振动预测系统.docx
基于Matlab和BP神经网络的爆破振动预测系统1.IntroductionBlastingvibrationisanimportantfactorinminingengineering,constructionengineering,andgeologicalengineering.Itsimpactontheenvironmentcannotbeignored.Inordertoreducetheimpactofblastingvibration,itisnecessarytoaccuratelypr
基于MATLAB的BP神经网络预测系统的设计.docx
基于MATLAB的BP神经网络预测系统的设计基于MATLAB的BP神经网络预测系统的设计摘要:神经网络在预测系统中具有广泛的应用。本文设计一个基于MATLAB的BP神经网络预测系统,用于对未知数据进行预测。首先介绍了BP神经网络的原理和结构,然后详细说明了系统设计的各个环节,包括数据预处理、网络拓扑结构的确定、权值初始化、训练和测试等。最后,通过真实数据的测试,验证了该系统的预测性能和准确性。关键词:BP神经网络;预测系统;MATLAB;数据预处理;网络拓扑结构一、引言神经网络是模拟人脑神经系统工作原理的
基于BP神经网络模型的爆破对邻近管道振动预测研究.docx
基于BP神经网络模型的爆破对邻近管道振动预测研究标题:基于BP神经网络模型的爆破对邻近管道振动预测研究摘要:爆破对邻近管道振动的预测是管道设计与安全性评估中重要的一环。本研究以BP神经网络模型为基础,针对爆破对邻近管道振动影响进行了预测研究。建立了合适的训练数据集,并进行了神经网络模型的训练与优化。通过比较模型的预测结果与实际测量数据,验证了BP神经网络的有效性和准确性。本研究的结果对管道工程的设计与安全性评估具有一定的指导意义。1.引言随着经济的发展和城市化进程的加快,管道工程在城市建设中的地位变得越来
基于遗传BP神经网络的煤矿爆破振动特征参量预测.docx
基于遗传BP神经网络的煤矿爆破振动特征参量预测煤矿爆破振动特征参量预测的研究一直是煤矿行业中的重要课题之一。煤矿爆破振动对矿山周围环境的影响较大,因此准确预测爆破振动特征参量对于保证矿山安全和生态环境的稳定具有重要意义。传统的预测方法存在一些问题,例如模型复杂度高、预测精度低等,因此需要一个新的方法来提高预测精度。近年来,神经网络的发展已经取得了许多突破性的成果。BP神经网络是其中一种常用的神经网络模型,它可以通过调整连接权重来建立非线性映射关系。然而,BP神经网络在处理复杂数据时存在容易陷入局部最优、训
基于BP神经网络模型的爆破对邻近管道振动预测研究.docx
基于BP神经网络模型的爆破对邻近管道振动预测研究基于BP神经网络模型的爆破对邻近管道振动预测研究摘要:爆破是一种常见的工业作业方式,在一些领域中广泛应用,但爆破过程会产生较大的震动,对邻近管道的振动造成潜在的威胁。为了预测爆破对邻近管道的振动影响,本研究基于BP神经网络模型进行了相关研究。通过收集现有的管道振动数据并建立训练集和测试集,利用BP神经网络模型进行训练和测试,最后得到了较为准确的预测结果。研究结果表明,基于BP神经网络模型的爆破对邻近管道振动预测具有较高的准确性和可靠性。关键词:爆破,邻近管道