
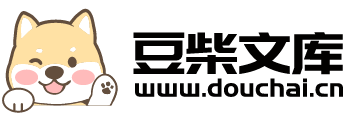
交叉反向学习和同粒社会学习的粒子群优化算法.docx
骑着****猪猪
在线预览结束,喜欢就下载吧,查找使用更方便
相关资料
交叉反向学习和同粒社会学习的粒子群优化算法.docx
交叉反向学习和同粒社会学习的粒子群优化算法ParticleSwarmOptimization(PSO)isameta-heuristicalgorithminspiredbythesocialbehaviorofbirdflocksandfishschools.PSOhasbeenwidelyusedinoptimizationproblemsduetoitssimplicityandeffectivenessinfindingoptimalsolutions.However,likeothermeta-
自适应精英反向学习的粒子群优化算法.docx
自适应精英反向学习的粒子群优化算法标题:自适应精英反向学习的粒子群优化算法摘要:粒子群优化算法(PSO)是一种经典的优化算法,其通过模拟鸟群捕食行为来寻找最优解。然而,传统的PSO算法在应用于复杂优化问题时,往往存在收敛速度慢、易陷入局部最优等问题。为了克服这些问题,本文提出了一种自适应精英反向学习的粒子群优化算法(AEL-PSO),通过引入自适应学习和反向学习机制,提高算法搜索能力与收敛速度。实验结果表明,AEL-PSO算法在常见优化问题求解中具有更好的性能。第一部分:引言1.1研究背景1.2文章结构第
一种融合反向学习和量子优化的粒子群算法.docx
一种融合反向学习和量子优化的粒子群算法融合反向学习和量子优化的粒子群算法摘要:粒子群算法是一种基于群体智能的优化算法,具有全局搜索和收敛性好等优点。然而,传统的粒子群算法在处理复杂的高维问题时存在搜索空间大、易陷入局部最优等问题。为了克服这些问题,本文提出了一种新的融合反向学习和量子优化的粒子群算法。该算法引入了反向学习的思想,利用已有的知识来指导粒子的搜索行为。同时,通过引入量子优化的技术,将粒子群算法的搜寻过程变得更加精确和高效。实验结果表明,融合了反向学习和量子优化的粒子群算法在解决高维复杂问题时具
一种精英反向学习的粒子群优化算法.docx
一种精英反向学习的粒子群优化算法粒子群优化算法是一种基于群体智能的优化算法,它模拟了鸟群或鱼群等生物群体的行为,并通过合作与竞争的机制,逐步优化解空间中的目标函数值。粒子群优化算法经过多年的发展,已经在各种优化问题上得到了广泛的应用。然而,在某些特定的问题上,传统的粒子群优化算法可能存在性能不佳的问题。为了解决这一问题,研究者提出了一种精英反向学习的粒子群优化算法。精英反向学习的粒子群优化算法是一种能够充分利用已知最优解信息的算法。该算法通过引入精英个体,将它们的经验知识传递给其他粒子,并指导粒子群的搜索
一种邻域重心反向学习的粒子群优化算法.docx
一种邻域重心反向学习的粒子群优化算法标题:一种邻域重心反向学习的粒子群优化算法摘要:在优化问题的解空间中,粒子群优化算法(ParticleSwarmOptimization,PSO)是一种基于群体智能的全局优化算法。传统的PSO算法在解决复杂问题时,容易陷入局部最优解,导致性能下降。为了解决这个问题,本文提出了一个改进的PSO算法,被称为邻域重心反向学习的粒子群优化算法(RetrospectiveLearningbasedNeighborhoodCentroidParticleSwarmOptimizat