
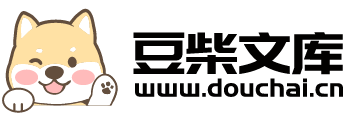
基于主题本体扩展特征的短文本分类.docx
快乐****蜜蜂
在线预览结束,喜欢就下载吧,查找使用更方便
相关资料
基于主题本体扩展特征的短文本分类.docx
基于主题本体扩展特征的短文本分类摘要短文本分类是自然语言处理的重要领域之一,它对于信息检索和文本分类具有重要的意义。在短文本分类过程中,主题本体能够对文本特征进行有效的扩展,提高文本分类的准确率和性能。本文对于基于主题本体扩展特征的短文本分类技术进行了研究和探讨。通过实验验证,证明主题本体扩展特征在短文本分类中具有重要的应用价值。本文介绍了基于主题本体扩展特征的短文本分类技术的基本原理和应用方法,分析了国内外研究现状和存在问题,并提出了未来的研究方向和展望。关键词:短文本分类;主题本体;特征扩展;文本分类
基于主题模型与深度学习的短文本特征扩展与分类研究.docx
基于主题模型与深度学习的短文本特征扩展与分类研究基于主题模型与深度学习的短文本特征扩展与分类研究摘要:随着社交媒体和互联网的快速发展,人们生成了大量的短文本数据,这些短文本不但具有信息量大、表达方式多样等特点,同时也带来了文本特征表示的挑战。传统的词袋模型往往忽略了短文本的上下文信息,因此短文本的特征表示和分类面临着很大的挑战。针对这个问题,本文提出了一种基于主题模型和深度学习的短文本特征扩展与分类的方法。该方法首先利用主题模型提取短文本的主题信息,然后利用深度学习技术对主题信息进行特征表示和分类。实验证
基于主题模型与深度学习的短文本特征扩展与分类研究的开题报告.docx
基于主题模型与深度学习的短文本特征扩展与分类研究的开题报告一、选题背景随着社会信息化发展,人们发布的信息变得越来越多,而且这些信息往往是以短文本的形式存在。但是,短文本由于信息量相对较少,可能会存在分类精度低、文本特征稀疏等问题。为了有效应对这些问题,目前一些研究正在将主题模型和深度学习结合在一起,以便扩展短文本的特征并提升其分类精度。二、研究目的和意义本课题旨在通过探究主题模型和深度学习相结合的方法,对短文本的特征进行扩展,提升其分类精度和准确性。该研究对于推进机器学习算法的发展和应用、提升短文本处理水
基于主题模型与深度学习的短文本特征扩展与分类研究的任务书.docx
基于主题模型与深度学习的短文本特征扩展与分类研究的任务书任务书一、任务背景随着网络技术的普及和应用,人们越来越多地通过网络进行文本信息的获取和交流。然而,网络上生成的文本短小精悍,往往仅有数十甚至数百字,面对这种短文本的分类任务,传统的机器学习方法往往面临着准确性与完整性的瓶颈。因此,如何有效地从短文本中提取特征,并对其进行分类,成为当前研究的重点。近年来,随着自然语言处理技术的发展,主题模型成为短文本特征提取的重要方法之一。主题模型是一种基于概率统计的文本建模方法,能够将文本表示为若干个主题的分布,从而
基于非负矩阵分解的短文本特征扩展与分类.docx
基于非负矩阵分解的短文本特征扩展与分类基于非负矩阵分解的短文本特征扩展与分类摘要:随着社交媒体的飞速发展,短文本数据在互联网中占据了重要的地位。然而,由于短文本数据的特点,传统的分类方法表现不佳。本文提出了一种基于非负矩阵分解的短文本特征扩展与分类方法。首先,我们通过非负矩阵分解技术将短文本数据表示为词袋模型。然后,我们使用主题模型对短文本数据进行特征扩展。最后,我们采用支持向量机分类器对扩展后的特征进行分类。实验结果表明,我们提出的方法在短文本分类任务上表现出色。关键词:短文本分类,非负矩阵分解,特征扩