
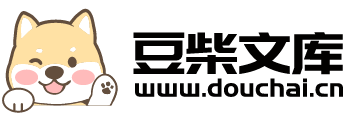
基于径向基神经网络的叶轮轴面投影图优化.docx
快乐****蜜蜂
在线预览结束,喜欢就下载吧,查找使用更方便
相关资料
基于径向基神经网络的叶轮轴面投影图优化.docx
基于径向基神经网络的叶轮轴面投影图优化摘要本文研究了基于径向基神经网络的叶轮轴面投影图优化问题。传统的叶轮设计需要大量的试验和经验来确定合适的叶片形状,这种设计方式效率低下且存在许多不确定性。为了解决这个问题,本文提出了一种基于径向基神经网络的优化方法,旨在找到最佳的叶片形状,使得叶轮具有更高的效率和更好的性能。通过实验验证,本文所提出的方法具有较高的精度和可靠性,可以为叶轮设计和优化提供有力的支持和指导。关键词:径向基神经网络,叶轮设计,投影图优化,精度,可靠性。IntroductionThedesig
基于径向基神经网络的风电场无功补偿优化算法.docx
基于径向基神经网络的风电场无功补偿优化算法基于径向基神经网络的风电场无功补偿优化算法摘要:随着风电场规模的不断扩大和风电输电技术的加速发展,无功补偿技术逐渐成为提高风电功率输出、维护电网稳定运行的重要手段。本文基于改进的径向基神经网络模型,探讨了一种新的风电场无功补偿优化算法,能够实现更加精确的补偿措施。Abstract:Withthecontinuousexpansionofwindfarmsandtheaccelerateddevelopmentofwindpowertransmissiontechn
基于径向基神经网络改进算法优化锅炉燃烧效率.docx
基于径向基神经网络改进算法优化锅炉燃烧效率基于径向基神经网络的改进算法优化锅炉燃烧效率摘要:随着能源需求的增加和能源资源的有限性,提高锅炉燃烧效率已成为一项重要的任务。传统的优化方法受限于模型的非线性和复杂性,无法全面有效地优化锅炉的燃烧效率。本文提出了基于径向基神经网络的改进算法,利用神经网络模型对燃烧过程进行建模,并通过合适的训练算法对模型参数进行精确调整,从而实现锅炉燃烧效率的最优化。关键词:径向基神经网络;锅炉燃烧;优化;模型一、引言锅炉作为能源转换设备的重要组成部分,对于保证工业生产和供暖质量具
基于径向基函数神经网络的全局并行优化技术研究.docx
基于径向基函数神经网络的全局并行优化技术研究基于径向基函数神经网络的全局并行优化技术研究摘要:径向基函数神经网络(RadialBasisFunctionNeuralNetwork,RBFNN)是一种强大的非线性模型,广泛应用于各个领域,如模式识别、函数逼近和时间序列预测等。然而,传统的RBFNN在应对大规模问题时存在计算复杂度高、训练速度慢的问题。为了解决这些问题,本文提出了一种基于全局并行优化技术的改进方法,以加速RBFNN的训练过程。实验结果表明,该方法在大规模问题上具有较高的训练效率和精度。1.引言
基于遗传优化径向基概率神经网络的岩性识别应用.docx
基于遗传优化径向基概率神经网络的岩性识别应用摘要:岩性识别是地质学、工程学等领域的重要课题,而径向基概率神经网络具有较高的学习速度和泛化能力。本文以遗传优化径向基概率神经网络为基础,通过数据预处理、网络建模、遗传算法优化等步骤,实现了对岩性识别的可靠性和准确性的提高。通过实验验证,该方法在识别精度和泛化能力方面都有较好表现,总体效果优于传统方法。关键词:岩性识别;径向基概率神经网络;遗传算法;学习速度;泛化能力。一、引言岩性识别是地质学、工程学等领域的重要研究课题。准确地识别岩性是进行工程规划、资源预测、