
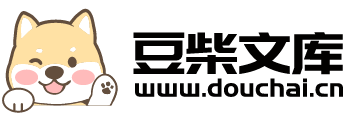
基于梯度提升决策树的高速公路行程时间预测模型(英文).docx
快乐****蜜蜂
在线预览结束,喜欢就下载吧,查找使用更方便
相关资料
基于梯度提升决策树的高速公路行程时间预测模型(英文).docx
基于梯度提升决策树的高速公路行程时间预测模型(英文)Title:PredictingHighwayTravelTimewithGradientBoostingDecisionTreesAbstract:Highwaytraveltimepredictionhasvitalimplicationsfortransportationmanagement,routeplanning,anddriverdecision-making.Thispaperproposesapredictivemodelforhigh
基于梯度提升决策树的高速公路交织区汇入模型.docx
基于梯度提升决策树的高速公路交织区汇入模型基于梯度提升决策树的高速公路交织区汇入模型摘要:高速公路交织区是城市交通网络中一种非常重要的路段,在车流量大、车辆通行速度高的情况下,其交通管理的复杂性和困难性增加。本论文提出了一种基于梯度提升决策树的高速公路交织区汇入模型,旨在提高交织区的交通效率和安全性。通过使用梯度提升决策树算法进行数据挖掘和模型训练,结合交织区的实际情况和限制条件,建立了汇入模型,并通过实验验证其有效性和可行性。关键词:高速公路,交织区,梯度提升决策树,数据挖掘,交通效率,安全性1.引言高
基于ARMAX模型的高速公路行程时间预测研究.docx
基于ARMAX模型的高速公路行程时间预测研究随着城市化进程的不断发展,交通问题越来越引起人们的关注。高速公路作为连接城市的重要交通枢纽,其行程时间的准确预测对于交通运输的管理和规划至关重要。因此,本文研究了基于ARMAX模型的高速公路行程时间预测方法。一、ARMAX模型的概述ARMAX模型是一种统计学方法,主要用于时间序列预测。它基于自回归过程、滑动平均过程和外生变量之间的关系来预测未来的值。在ARMAX模型中,自回归项是过去的观测值与对应的滞后观测值之间的关系,而滑动平均项则是过去的观测值与对应的滞后误
基于SV模型的行程时间预测.docx
基于SV模型的行程时间预测在日常生活中,人们经常需要制定出行计划,而行程的时间预测是其中关键的一环。行程时间预测的准确性直接影响出行计划的实施效果,因此行程时间预测是非常重要的。现今,基于SV模型的行程时间预测已经被应用到了现实生活中,并且已经得到了广泛的应用。本文将从SV模型的原理、建模的方法、以及实际应用等方面进行探讨和分析。一、SV模型的原理SV模型(SupportVectorMachine)是一种基于监督学习的模型。其基本思想是从数据集中找出一个最优分界面,将数据最佳地划分为两类。这个最优分界面就
基于梯度提升决策树集成模型的短时交通流量预测的开题报告.docx
基于梯度提升决策树集成模型的短时交通流量预测的开题报告一、研究背景和意义城市交通拥堵一直是城市发展中存在的难题,交通流量预测作为交通控制与规划的重要工具,对于优化城市交通运输及提高城市人员出行的效率有着重要意义。随着云计算、大数据等新技术的发展,交通流量预测的数据来源变得更加丰富,同时也促进了交通流量预测模型的发展。本研究旨在应用机器学习的方法,基于梯度提升决策树集成模型,构建一个短时交通流量预测模型,实现精准的交通流量预测,为城市交通规划优化和交通设计提供科学依据和参考。二、研究内容和工作计划1.研究现