
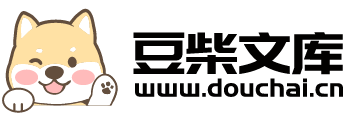
基于改进VGG卷积神经网络的前方车辆目标检测.docx
快乐****蜜蜂
在线预览结束,喜欢就下载吧,查找使用更方便
相关资料
基于改进VGG卷积神经网络的前方车辆目标检测.docx
基于改进VGG卷积神经网络的前方车辆目标检测Title:ImprovingVehicleObjectDetectionUsingEnhancedVGGConvolutionalNeuralNetworkAbstract:Vehicleobjectdetectionplaysavitalroleinautonomousdriving,advanceddriverassistancesystems(ADAS),andtrafficmonitoring.Inthispaper,weproposeanenhanc
基于改进VGG卷积神经网络的棉花病害识别模型.docx
基于改进VGG卷积神经网络的棉花病害识别模型摘要:随着棉花产量的增加,棉花病害的防治变得越来越重要。本论文提出了一种基于改进VGG卷积神经网络的棉花病害识别模型。通过对原始VGG网络的改进,我们提高了模型的性能,并成功地实现了对棉花病害的准确识别。在实验中,我们使用了一个包含大量不同类型棉花病害的数据集,并将其分为训练集和测试集。通过在训练集上训练模型,并在测试集上进行验证,我们得到了很好的实验结果。实验结果表明,我们的模型在棉花病害识别方面具有很好的性能,能够准确识别不同类型的棉花病害。本研究为棉花产业
基于改进VGG卷积神经网络的奶牛牛脸识别研究.docx
基于改进VGG卷积神经网络的奶牛牛脸识别研究标题:基于改进VGG卷积神经网络的奶牛牛脸识别研究摘要:奶牛的识别对于农牧业管理至关重要。传统的奶牛识别方法通常依赖手工设计的特征提取器和分类器,这些方法在面对复杂的奶牛牛脸图像时表现欠佳。为此,本文提出了一种基于改进的VGG卷积神经网络的奶牛牛脸识别方法。通过对原始VGG网络进行优化和改进,我们实现了更高效、准确的奶牛牛脸识别。实验结果表明,本文提出的方法在奶牛牛脸识别任务中取得了显著的性能提升。1.引言奶牛牛脸识别在农牧业管理中具有广泛的应用价值,可以帮助农
基于改进的卷积神经网络的车辆停车压线检测.docx
基于改进的卷积神经网络的车辆停车压线检测车辆停车压线检测在交通管理和场地安全方面起着重要的作用。然而,由于车辆停车时的复杂性和场景的多样性,传统的图像处理技术往往无法准确地进行停车压线检测。为了解决这一问题,本论文提出了一种基于改进的卷积神经网络的车辆停车压线检测方法。首先,介绍了传统的车辆停车压线检测方法的局限性。传统的方法通常基于特征提取和机器学习技术,如边缘检测、色彩分割和形态学操作等。然而,这些方法往往对于场景中的噪声和复杂性很敏感,且需要手动选择特征和调整参数,不够自适应和鲁棒。因此,需要一种更
基于改进的几何约束算法与卷积神经网络的车辆检测.docx
基于改进的几何约束算法与卷积神经网络的车辆检测基于改进的几何约束算法与卷积神经网络的车辆检测摘要:车辆检测是计算机视觉领域的关键任务之一,其在智能交通和自动驾驶等应用中具有重要的作用。传统的检测方法通常依赖于手工设计的特征和分类器,而近年来深度学习技术的发展使得卷积神经网络(CNN)成为车辆检测的主要方法之一。然而,传统的CNN方法往往不能很好地处理车辆的几何约束,导致检测结果不够准确。针对这一问题,本文提出了一种基于改进的几何约束算法与卷积神经网络的车辆检测方法。关键词:车辆检测、几何约束、卷积神经网络