
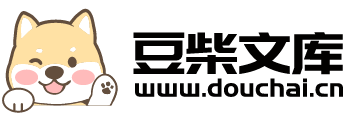
基于改进BBO算法优化KELM的短期风电功率预测.docx
快乐****蜜蜂
在线预览结束,喜欢就下载吧,查找使用更方便
相关资料
基于改进BBO算法优化KELM的短期风电功率预测.docx
基于改进BBO算法优化KELM的短期风电功率预测Title:Short-TermWindPowerPredictionBasedonImprovedBBOAlgorithmOptimizingKELMAbstract:Accuratelypredictingwindpowergenerationiscrucialforefficientintegrationandutilizationofrenewableenergysources.Thispaperproposesanimprovedapproacht
基于改进小波包算法的风电功率短期预测.docx
基于改进小波包算法的风电功率短期预测摘要风能作为一种清洁、可再生的能源,在现代社会中得到了广泛的应用。由于风速的不稳定性和不确定性,风电功率的预测对于风电场的运行管理和电力市场交易具有重要意义。本文基于改进小波包算法,对风电功率进行了短期预测。首先,对于实际的风电功率数据进行了预处理和特征提取;其次,采用小波包分解技术对原始数据进行分解预测,通过对小波包系数的选择和阈值的调整,提高了预测精度。最后,通过对比实验,证明了本文所提出的改进小波包算法对于风电功率预测的有效性和优越性。关键词:风电功率预测;小波包
基于改进引力搜索算法的风电功率短期预测优化调度研究.docx
基于改进引力搜索算法的风电功率短期预测优化调度研究摘要风电功率的短期预测在风电场的运营和调度中起着关键作用。为了提高风电功率的预测精度和优化调度效果,本文提出了一种基于改进引力搜索算法的风电功率短期预测优化调度方法。首先,分析了风电功率预测的重要性和优化调度的挑战。然后,介绍了引力搜索算法及其在优化问题中的应用。接着,针对风电功率预测和优化调度问题的特点,对引力搜索算法进行了改进,包括引入自适应参数控制策略和精英策略。最后,在风电场的实际数据上进行了实验验证。实验结果表明,本文提出的方法能够显著提高风电功
基于改进TLBO优化LSSVM的风电功率短期预测.docx
基于改进TLBO优化LSSVM的风电功率短期预测基于改进TLBO优化LSSVM的风电功率短期预测摘要:风力发电作为可再生能源的重要形式之一,其功率预测对于风电场的运行和管理至关重要。本文基于改进的Teaching-Learning-BasedOptimization(TLBO)算法,结合LeastSquaresSupportVectorMachine(LSSVM)模型,提出了一种改进TLBO优化LSSVM的风电功率短期预测方法。通过对该方法进行了不同风电场数据集的测试,验证了其在风力发电功率预测中的有效性
基于EWT-KELM方法的短期风电功率组合预测.docx
基于EWT-KELM方法的短期风电功率组合预测基于EWT-KELM方法的短期风电功率组合预测摘要风力发电是一种可再生的清洁能源,随着其在电力系统中的比例增加,风电功率预测的准确性对于电力系统的稳定运行和调度具有重要意义。本论文提出了一种基于经验小波变换(EWT)和核极限学习机(KELM)方法的短期风力发电功率组合预测模型。该模型结合了EWT的多尺度分析能力和KELM的高效计算能力,能够更好地捕捉风电功率时间序列的非线性特征。通过对中国某风电场的实际风速和风电功率数据进行实证分析,结果表明该方法在短期风电功