
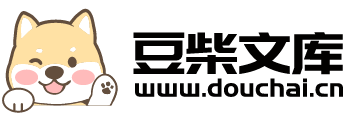
Ensemble-SISPLS近红外光谱变量选择方法.docx
快乐****蜜蜂
在线预览结束,喜欢就下载吧,查找使用更方便
相关资料
Ensemble-SISPLS近红外光谱变量选择方法.docx
Ensemble-SISPLS近红外光谱变量选择方法Abstract:Ensemble-SISPLS(SequentialImportanceSamplingPartialLeastSquares)isafeatureselectionmethodthatcombinesEnsembleLearningandPartialLeastSquares(PLS)regressionfornear-infrared(NIR)spectraldata.Inthispaper,weintroducetheEnsemb
基于灰狼算法的近红外光谱变量选择方法研究.docx
基于灰狼算法的近红外光谱变量选择方法研究基于灰狼算法的近红外光谱变量选择方法研究摘要:近红外光谱技术已经成为了无损检测和分析的一种重要手段。然而,近红外光谱数据庞大且复杂,数据中包含大量无关变量,会降低建模和检测的效率。因此,选择对建模和检测有重要影响的有效变量进行分析和优化是一个关键问题。本文提出了一种基于灰狼算法的近红外光谱变量选择方法,通过灰狼算法对近红外光谱数据进行优化的变量选择,提高建模和检测的效率。实验结果表明,本文提出的方法能够较好地从近红外光谱数据中选择出具有重要影响的变量,提高建模和检测
近红外光谱建模中的变量选择方法研究的开题报告.docx
近红外光谱建模中的变量选择方法研究的开题报告一、选题背景近红外光谱技术(NIR)是近年来发展迅速的一种分析技术,已经广泛地应用于农业、食品、化学、制药及医学等领域。NIR光谱技术通过检测样品中物质的振动与转动,可得到其特征光谱,从而实现定量或定性的分析和检测。为了达到更好的分析效果,需要对样本进行预处理和变量选择等操作。其中,变量选择是NIR光谱建模的重要环节,直接影响模型的可靠性和预测精度。因此,研究NIR光谱的变量选择方法具有重要意义。二、研究目的和内容本文旨在研究近红外光谱建模中的变量选择方法,探究
一种基于LASSO的近红外光谱变量选择方法.pdf
一种基于LASSO的近红外光谱变量选择方法,具体过程如下:采集样本的近红外光谱,用常规方法测定被测成分浓度向量;采用一定的分组方式将数据集分成训练集和预测集;采用交叉验证确定LASSO方法的约束值t;利用最小角回归算法计算回归系数β,保留β不为0的波长点的位置;利用保留的波长对应的训练集光谱与浓度向量间建立偏最小二乘回归模型,对预测集样本被测成分浓度进行预测。该方法能提取出有效波长,简化了定量分析模型,提高了模型的预测精度。与已有变量选择方法相比,具有快速、可重复、用更少的变量数达到更高预测精度的优势。本
近红外光谱建模中的变量选择方法研究的任务书.docx
近红外光谱建模中的变量选择方法研究的任务书一、选题背景近红外(NIR)光谱技术是一种非破坏性快速分析技术,具有广泛的应用领域,例如食品、医药、农产品、环境等。在NIR光谱建模中,变量选择是一个关键问题,它直接影响模型的精度和稳定性。变量选择方法的优劣直接决定了模型的预测精度和稳定性,因此为进一步提高NIR建模的效果,有必要对变量选择方法进行研究。二、研究目的此次研究的目的是探索有效的变量选择方法来优化NIR建模结果。具体目标如下:1.系统梳理目前常用的变量选择方法,包括Univariate,PCA等方法,