
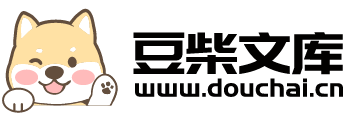
基于图像处理的连铸方坯低倍缺陷裂纹和缩孔的识别与评级.docx
快乐****蜜蜂
在线预览结束,喜欢就下载吧,查找使用更方便
相关资料
基于图像处理的连铸方坯低倍缺陷裂纹和缩孔的识别与评级.docx
基于图像处理的连铸方坯低倍缺陷裂纹和缩孔的识别与评级摘要连铸是现代钢铁生产中重要的工艺之一,方坯是连铸的主要产品之一。然而,方坯在生产过程中常常会出现缺陷,如裂纹和缩孔等。这些缺陷不仅会降低产品的质量,还可能对后续加工和使用产生不良影响。因此,开发一种图像处理的方法来识别和评级方坯中的低倍缺陷,对提高产品质量和生产效率具有重要意义。本文通过对连铸方坯进行数字图像采集并进行预处理,包括图像去噪、图像增强等,以提高后续缺陷识别的准确性。然后,采用特征提取的方法,提取方坯图像中的关键特征,如纹理、形状等。通过建
基于图像处理的连铸方坯低倍缺陷裂纹和缩孔的识别与评级的开题报告.docx
基于图像处理的连铸方坯低倍缺陷裂纹和缩孔的识别与评级的开题报告一、研究意义及背景钢铁生产领域的连铸是一项重要的生产工艺。然而在连铸过程中,方铸坯往往会出现一些缺陷,如裂纹和缩孔等,这些缺陷会降低方铸坯的质量,影响钢板的性能和质量。因此,为了保证钢板的质量和市场竞争力,对连铸方坯的缺陷进行及时准确地识别和评级是非常必要的。在过去,这种识别和评级通常是依赖人工操作完成的,但是这种劳动力和时间成本高,效率低,并且由于人为的主观因素,诊断结果也存在偏差。因此,开发一种基于图像处理的连铸方坯低倍缺陷识别与评级的新方
基于图像处理的连铸方坯低倍缺陷裂纹和缩孔的识别与评级的任务书.docx
基于图像处理的连铸方坯低倍缺陷裂纹和缩孔的识别与评级的任务书任务书一、任务背景在连铸方坯生产过程中,方坯的质量直接影响到后续的加工工艺和产品质量。连铸方坯存在着各种各样的表面缺陷,其中低倍缺陷包括了表面裂纹和缩孔。这些缺陷会影响方坯的加工性能和强度,甚至会导致产品质量问题。因此,对连铸方坯的低倍缺陷进行精确的识别和评级,对于生产管理和产品质量的控制非常重要。基于图像处理的技术应用于连铸方坯的表面缺陷识别和评级已经得到了广泛的应用。图像处理技术可以对方坯的表面图像进行处理,提取出其中的缺陷信息,进行缺陷检测
基于图像处理的连铸方坯低倍缺陷中心偏析与裂纹的识别与评级的开题报告.docx
基于图像处理的连铸方坯低倍缺陷中心偏析与裂纹的识别与评级的开题报告一、课题背景现代钢铁生产中,连铸方坯是重要的半成品,其质量对后续轧制加工、制品质量和使用寿命等具有直接影响。方坯品质不良或存在缺陷将导致制品批量退货、钢材利用率下降,进而影响整个钢铁产业的发展。目前,钢铁企业普遍存在连铸方坯质量管理欠缺的问题,缺乏有效的方案和手段来提升连铸方坯的质量。因此,研究连铸方坯低倍缺陷中心偏析与裂纹的识别与评级,对于提高连铸方坯质量具有重要的意义。二、研究内容本文研究的核心内容是连铸方坯低倍缺陷中心偏析与裂纹的识别
连铸坯低倍中心裂纹分析与探讨.docx
连铸坯低倍中心裂纹分析与探讨标题:连铸坯低倍中心裂纹分析与探讨摘要:连铸坯是制备金属材料的重要工艺过程。然而,连铸坯在制备过程中容易出现中心裂纹,降低了产品的质量和使用寿命。本论文将从连铸坯低倍中心裂纹的形成机理进行分析,并探讨其影响因素与防控措施。通过对连铸坯低倍中心裂纹的研究,可以进一步提高连铸坯制备的质量和稳定性。关键词:连铸坯、中心裂纹、形成机理、影响因素、防控措施1.引言连铸坯作为金属材料制备中的重要工艺过程,尤其在钢铁行业中具有重要的应用。然而,在连铸坯制备过程中,常常出现中心裂纹的问题,导致