
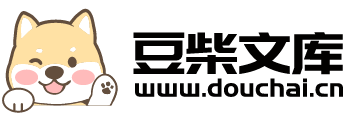
基于DenseNet的单目图像深度估计.docx
快乐****蜜蜂
在线预览结束,喜欢就下载吧,查找使用更方便
相关资料
基于DenseNet的单目图像深度估计.docx
基于DenseNet的单目图像深度估计Title:SingleImageDepthEstimationBasedonDenseNetAbstract:DepthestimationisacriticaltaskincomputervisionthataimstoestimatethedepthinformationofascenefromasingleRGBimage.Thispaperproposesanovelapproachforsingleimagedepthestimation,combinin
基于深度学习的单目图像深度估计.docx
基于深度学习的单目图像深度估计基于深度学习的单目图像深度估计摘要:深度图像是计算机视觉领域中一个重要的问题,它在许多领域中发挥着重要的作用,如三维重建、物体检测和跟踪等。传统的方法通常依赖于多个相机或者深度传感器来获取深度信息。然而,这种方法通常成本昂贵且不便于应用,因此,单目图像深度估计成为一个热门的研究方向。近年来,深度学习的快速发展为单目图像深度估计提供了新的机会。本文将综述基于深度学习的单目图像深度估计的研究现状和发展趋势,并讨论其中的挑战和解决方案。1.引言深度图像是指每个像素点相对于相机的距离
基于单目图像的人脸深度估计.docx
基于单目图像的人脸深度估计随着计算机视觉技术的不断发展,人们越来越需要能够准确估计人脸深度的算法。而基于单目图像的人脸深度估计算法是其中一种重要的技术。本文将对单目图像人脸深度估计技术作一个简要的综述。一、引言人脸深度估计是计算机视觉中的一个重要任务,它主要应用于身份验证、虚拟现实、医学研究等领域。目前,采用多种传感器的多个方法用于人脸深度估计,但这些方法通常需要高成本的硬件或复杂的算法。单目图像人脸深度估计技术则可以在仅使用单目图像的情况下获得人脸深度信息,是一种代价较低、易用性较高的方法,具有很大的研
单目图像的深度估计.docx
单目图像的深度估计标题:单目图像的深度估计摘要:深度估计是计算机视觉领域一个重要的任务,在许多应用中都有着广泛的应用。本论文将重点探讨单目图像的深度估计方法及其应用。首先介绍深度估计的研究背景和意义,然后分析单目图像深度估计的挑战和问题。接着,介绍基于机器学习的单目图像深度估计方法,包括传统的基于特征的方法和基于深度学习的方法。随后,讨论了单目图像深度估计的应用领域,并对目前存在的问题和未来的研究方向进行了展望。1.引言1.1研究背景和意义深度信息是场景理解和场景感知的重要组成部分,对于许多计算机视觉任务
基于深度学习的单目图像深度估计的开题报告.docx
基于深度学习的单目图像深度估计的开题报告一、选题背景单目图像深度估计是计算机视觉中的重要问题,它能够为自动驾驶、机器人导航以及虚拟现实等领域提供基础和支撑。传统的深度估计方法多依赖于手工设计的特征和先验知识,而深度学习技术的广泛应用使得基于数据驱动的单目图像深度估计成为可能。随着深度学习技术的不断发展,卷积神经网络(ConvolutionalNeuralNetwork,CNN)在深度估计中的应用也不断得到完善和拓展。CNN已经成为处理图像数据的重要工具,它可以对图像数据进行端到端的训练和直接推断,避免了对