
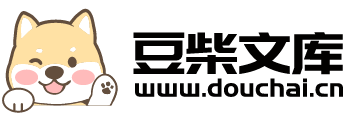
一种融合深度基于灰度共生矩阵的感知模型.docx
快乐****蜜蜂
在线预览结束,喜欢就下载吧,查找使用更方便
相关资料
一种融合深度基于灰度共生矩阵的感知模型.docx
一种融合深度基于灰度共生矩阵的感知模型Title:ACognitiveModelbasedontheFusionofDeepLearningandGrayLevelCo-occurrenceMatrixAbstract:Deeplearningtechniqueshaveachievedremarkablesuccessinavarietyofperceptiontasks,includingimageclassificationandobjectrecognition.However,theseappr
一种基于灰度共生矩阵模型的羊毛羊绒识别算法.pdf
一种基于灰度共生矩阵模型的羊毛羊绒识别算法,包括在线识别流程和模型学习流程,所述在线识别流程,包括:(1)对羊绒羊毛纤维进行取像;(2)采用高斯滤波器对图像进行平滑滤波;(3)采用基于canny的边缘检测与轮廓对图像目标提取;(4)计算纹理特征量,采用反差、能量、熵、相关性4种统计量来表示纹理特征;(5)将得到的特征向量直接作为输入特征向量;所述模型学习流程,包括:(1)积累大量羊毛羊绒数据库;(2)人工标注使机器明确目标纤维的种类与所处的位置;(3)对数据库中纤维图像进行预处理、特征提取;(4)采取三层
一种基于灰度共生矩阵的红外与可见光图像融合方法.pdf
本发明公开了一种基于灰度共生矩阵的红外与可见光图像融合方法。首先,对红外源图像进行灰度共生矩阵分析,得到红外目标显著图。其次,对可见光和红外源图像进行非下采样轮廓波变换(NSCT),对分解得到的低频子带图像进行保持对比度的融合,对高频子带图像采用改进的高斯差分方法进行融合;再将目标显著图映射至融合后的低频子带图像上。最后,进行NSCT逆变换得到最终的融合图像。本发明利用灰度共生矩阵的纹理分析特性进行红外目标显著性检测,能够有效地提取红外目标并保留丰富的细节信息,改善融合后图像的质量。本发明提出的方法的客观
基于灰度共生矩阵的信噪比图像检测.docx
基于灰度共生矩阵的信噪比图像检测一、引言:信噪比是表示图像质量的重要参数之一,许多图像处理与识别算法也需要依赖信噪比结果进行调整和测试,因此信噪比图像检测在图像处理中具有重要的作用。图像处理是对数字图像进行分析和处理的过程,而图像中的信号通常被淹没在噪声中,因此,为了正确处理图像数据,需要对图像信噪比进行检测。灰度共生矩阵是用于图像分析和处理的常用方法之一,本文将介绍如何使用灰度共生矩阵进行信噪比图像检测。二、灰度共生矩阵:灰度共生矩阵又叫灰度共生概率矩阵,它是描述图像中像素灰度分布模式的矩阵,旨在描述图
基于灰度共生矩阵的织物纹理研究.docx
基于灰度共生矩阵的织物纹理研究一、引言纺织品作为一种广泛的生产和消费产品,其纹理特征和物理属性对其质量和价值的影响非常大。随着纺织品生产技术的不断进步,人们对织物纹理的研究也越来越深入。织物纹理的表征方法千差万别,其中灰度共生矩阵是一种流行的描述方法,本文以此为基础,介绍了织物纹理研究的基本方法和应用。二、灰度共生矩阵灰度共生矩阵是一种用于描述数字图像纹理的方法,它通过比较图像中不同灰度级别之间的相对位置和出现频率来定义图像纹理的属性。具体来说,灰度共生矩阵是一个$N_g$x$N_g$的矩阵,其中$N_g