
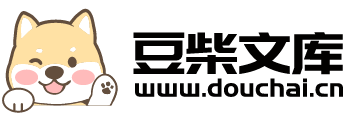
基于经验模态分解、多尺度熵算法和支持向量机的滚动轴承故障诊断方法.docx
快乐****蜜蜂
在线预览结束,喜欢就下载吧,查找使用更方便
相关资料
基于经验模态分解、多尺度熵算法和支持向量机的滚动轴承故障诊断方法.docx
基于经验模态分解、多尺度熵算法和支持向量机的滚动轴承故障诊断方法Title:FaultDiagnosisMethodforRollingBearingBasedonEmpiricalModeDecomposition,MultiscaleEntropyAlgorithm,andSupportVectorMachineAbstract:Rollingbearingsarewidelyusedinvariousindustrialmachinery,andtheirfaultscanleadtoserious
基于经验模态分解和支持向量机的滚动轴承故障诊断.docx
基于经验模态分解和支持向量机的滚动轴承故障诊断标题:基于经验模态分解和支持向量机的滚动轴承故障诊断摘要:滚动轴承是旋转机械中常用的元件之一,其故障会对机械设备的正常运行产生重大影响。传统的故障诊断方法往往只能通过直接监测振动信号进行故障判断,但由于振动信号存在噪声和非线性特征,诊断精度有限。因此,本文提出了一种基于经验模态分解(EMD)和支持向量机(SVM)的滚动轴承故障诊断方法,通过将振动信号分解成多个固有模态函数(IMF)并结合SVM实现故障的有效识别与分类。实验结果表明,该方法能够提高滚动轴承故障诊
基于经验模态分解和超球多类支持向量机的滚动轴承故障诊断方法.docx
基于经验模态分解和超球多类支持向量机的滚动轴承故障诊断方法摘要:针对滚动轴承故障诊断中信号复杂、特征难提取的问题,本文提出了一种基于经验模态分解和超球多类支持向量机的滚动轴承故障诊断方法。该方法首先使用经验模态分解(EMD)对滚动轴承振动信号进行分解,提取出信号的固有模态函数(IMF),并且针对不同IMF进行特征提取。然后,将提取出的IMF特征作为超球多类支持向量机(MCSVM)的输入,利用该分类器对不同故障种类进行分类识别,实现了滚动轴承的故障诊断。关键词:滚动轴承;故障诊断;经验模态分解;超球多类支持
基于精细复合多尺度散布熵与支持向量机的滚动轴承故障诊断方法.docx
基于精细复合多尺度散布熵与支持向量机的滚动轴承故障诊断方法摘要:滚动轴承是机械设备中一种常见的关键零部件,其故障会直接影响设备的性能和可靠性。因此,滚动轴承故障的诊断方法研究具有重要意义。本文提出一种基于精细复合多尺度散布熵与支持向量机的滚动轴承故障诊断方法。该方法首先使用小波分解对轴承振动信号进行多尺度分析,然后针对不同尺度下的子信号计算散布熵,从而提取故障特征。接着,利用支持向量机进行分类和诊断。通过实验验证,本文方法能够有效地实现滚动轴承故障的快速诊断,具有较高的准确性和稳定性。关键词:滚动轴承;故
基于变分模态分解排列熵和粒子群优化支持向量机的滚动轴承故障诊断方法.docx
基于变分模态分解排列熵和粒子群优化支持向量机的滚动轴承故障诊断方法摘要:随着滚动轴承在机械制造业中的广泛应用,如何有效地进行故障诊断是工程师们最为关心的问题。本文基于变分模态分解排列熵和粒子群优化支持向量机,提出了一种滚动轴承故障诊断方法。该方法通过对振动信号进行变分模态分解得到信号的主要频率和排列熵,再结合粒子群优化支持向量机对滚动轴承运行状态进行诊断。实验结果表明,本文提出的方法可以有效地诊断出滚动轴承故障,并且具有一定的鲁棒性和可靠性。关键词:滚动轴承;故障诊断;变分模态分解排列熵;粒子群优化支持向