
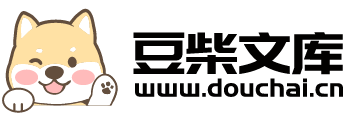
基于LM-BP神经网络的汽车AB柱内饰板注塑CAE优化分析.docx
快乐****蜜蜂
在线预览结束,喜欢就下载吧,查找使用更方便
相关资料
基于LM-BP神经网络的汽车AB柱内饰板注塑CAE优化分析.docx
基于LM-BP神经网络的汽车AB柱内饰板注塑CAE优化分析摘要随着汽车工业的快速发展,汽车内饰板注塑技术成为汽车制造的重要组成部分。该研究旨在基于LM-BP神经网络对汽车AB柱内饰板注塑过程进行优化分析,以提高生产效率和质量。通过CAE模拟软件建立模型,对注塑过程进行仿真计算,得出整体注塑参数和内饰板各部位的流量、温度、压力等数据。基于这些参数建立LM-BP神经网络,进行学习和训练,提高神经网络模型的准确性。然后使用改进的LM-BP神经网络进行参数优化分析,以减少内饰板出现缺陷和减少成本为目标,提高内饰板
基于CAE汽车B柱饰板变形分析及工艺优化研究.pptx
汇报人:目录PARTONEPARTTWO变形原因分析变形量计算变形模式分析变形对性能的影响PARTTHREECAE模拟技术介绍模拟过程与结果分析优化方案设计与验证优化效果评估与改进建议PARTFOUR工艺参数选择与调整实验设计与数据分析参数优化方案确定优化效果评估与改进建议PARTFIVE优化后B柱饰板性能测试测试结果分析与评价与传统方法的比较优化后性能的可持续性评估PARTSIX研究成果总结对未来研究的建议与展望THANKYOU
基于CAE技术的汽车上饰板注塑成型优化.docx
基于CAE技术的汽车上饰板注塑成型优化基于CAE技术的汽车上饰板注塑成型优化摘要:汽车上饰板作为车辆内部装饰的重要部件,注塑成型工艺对其质量和性能具有决定性影响。本文以一汽大众某车型中心上饰板为研究对象,利用计算机辅助工程(CAE)技术对其注塑成型过程进行优化。通过建立数值模型,计算了喷嘴温度、模具温度、注塑速度等参数对成型件热应力分布的影响,并通过优化设计探究了最佳的成型工艺参数。实验结果表明,利用CAE技术对汽车上饰板注塑成型进行优化可以有效提高产品质量,减少生产成本,具有重要的工程应用价值。关键词:
基于CAE和RBF神经网络的注塑工艺优化分析.docx
基于CAE和RBF神经网络的注塑工艺优化分析注塑工艺优化分析在现代注塑工业中起着重要的作用。通过对注塑工艺参数进行优化,可以提高注塑产品的质量、降低生产成本和提高生产效率。本文将介绍基于CAE(计算机辅助工程技术)和RBF(径向基函数)神经网络的注塑工艺优化分析。首先,我们将介绍了CAE技术在注塑工艺中的应用。CAE技术可以使用数学模型和计算机模拟来模拟注塑过程中的各种物理现象和工艺参数,如熔融流动、冷却和固化等。通过CAE技术,我们可以预测和优化注塑工艺参数,以实现最佳的注塑产品质量。CAE技术可以大大
汽车C立柱内饰板注塑模具.pdf
本实用新型公开了汽车C立柱内饰板注塑模具,设置有便于进行组装的前模,且前模的下表面连接有后模;包括:水路,设置于所述前模的内表面,且前模的下表面通过后模在其内部进行嵌套伸缩组件,并且伸缩组件的内表面伸缩连接有伸缩杆,同时伸缩杆的下表面螺纹组装有嵌套块。该汽车C立柱内饰板注塑模具,设置有嵌套在底膜中的进料嘴,可反方向注塑,并配合连接的主流道,以及主流道与分流道形成随型结构,便于根据随型浇口设计消除缩印,提高注塑效果,在主流道和分流道上设置加热丝,配合独立的线路接头单独控制不同的位置,进而设置的保温组件,有效