
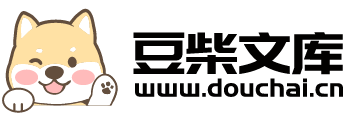
支持向量机的核方法研究及其在森林火灾视频识别中的应用.docx
快乐****蜜蜂
在线预览结束,喜欢就下载吧,查找使用更方便
相关资料
支持向量机的核方法研究及其在森林火灾视频识别中的应用.docx
支持向量机的核方法研究及其在森林火灾视频识别中的应用AbstractSupportVectorMachines(SVM)isamachinelearningalgorithmthathasbeenusedwidelyinvariousfieldssuchaspatternrecognition,textclassification,andimagerecognition.ThesuccessofSVMinmanyapplicationscanbeattributedtoitsexcellentperfor
支持向量机的核方法研究及其在森林火灾视频识别中的应用的中期报告.docx
支持向量机的核方法研究及其在森林火灾视频识别中的应用的中期报告一、研究背景和意义随着计算机视觉和大数据技术的发展,视频内容的自动分析和识别逐渐成为研究热点。在森林火灾的实时监测和控制中,视频监控系统可以实现对森林火灾的早期预警和快速响应。但是,森林火灾视频数据的复杂性和实时性对视频信号处理算法的准确性和实时性提出了挑战,进而需要开展相关的研究和解决。支持向量机(SVM)作为一种常用的分类器,已经在图像识别、语音识别等领域得到了广泛应用。而SVM的核方法更是使它在非线性分类问题上具有极高的准确性和泛化能力。
支持向量机在森林火灾识别中的应用研究.docx
支持向量机在森林火灾识别中的应用研究摘要森林火灾给人民生命财产带来重大威胁,因此在森林火灾的早期识别和有效控制方面,发展森林火灾识别技术尤为重要。本文旨在探讨支持向量机在森林火灾识别中的应用研究。首先,简述了支持向量机的原理以及其在分类领域的应用。其次,介绍了利用支持向量机算法实现森林火灾识别的过程。然后,基于实际数据,分析了支持向量机算法在森林火灾识别中的有效性。最后,总结了支持向量机在森林火灾识别中的优缺点,并对其未来的应用前景进行了展望。关键字:支持向量机;森林火灾;识别1.前言森林火灾是一种常见的
核矩阵近似的研究及其在支持向量机中的应用.docx
核矩阵近似的研究及其在支持向量机中的应用核矩阵近似的研究及其在支持向量机中的应用摘要:随着机器学习在各个领域的广泛应用,对于支持向量机(SupportVectorMachine,SVM)方法的改进和优化需求也越来越迫切。核方法是SVM中重要的数学基础,然而,在大规模数据集上使用传统的核方法往往会面临存储和计算复杂度的挑战。核矩阵近似技术作为一种解决方案,可以有效减少计算复杂度,并在理论和实践中取得了显著的成果。本文将重点介绍核矩阵近似的研究进展,以及其在支持向量机中的应用。1.引言支持向量机是一种经典的监
支持向量机核参数选择及其应用.pptx
,CONTENTS第一章第二章背景介绍支持向量机概述核参数选择的重要性第三章核函数定义常见核函数类型核函数选择依据第四章基于网格搜索的方法基于贝叶斯优化的方法基于启发式搜索的方法基于随机搜索的方法第五章实验数据集介绍实验设置与评估指标不同核参数选择方法的性能比较实验结果分析与讨论第六章案例一:手写数字识别案例二:文本分类案例三:图像识别案例四:推荐系统第七章研究成果总结工作不足与展望感谢您的观看