
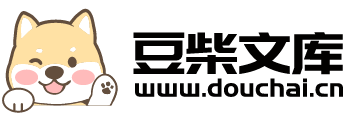
基于多特征的高空间分辨率遥感图像分类方法.docx
快乐****蜜蜂
在线预览结束,喜欢就下载吧,查找使用更方便
相关资料
基于多特征的高空间分辨率遥感图像分类方法.docx
基于多特征的高空间分辨率遥感图像分类方法摘要在高空间分辨率遥感图像分类中,多种地物和地貌也对分类结果产生影响。因此,本研究提出了一种基于多特征的高空间分辨率遥感图像分类方法,结合多种特征进行特征选择,使用支持向量机进行分类。实验结果表明,该方法在提高分类准确率的同时,能够提高分类效率,为高空间分辨率遥感图像分类提供了新的思路。关键词:高空间分辨率;遥感图像分类;多特征;支持向量机中文摘要在高空间分辨率遥感图像分类中,一张图中有多种地物和地貌也对分类结果产生影响,为此,本研究提出了一种基于多特征的高空间分辨
基于多种空间信息的高光谱遥感图像分类方法.docx
基于多种空间信息的高光谱遥感图像分类方法摘要高光谱遥感图像在土地利用、环境监测等领域具有广泛的应用,如何对高光谱遥感图像进行高效准确的分类是当前研究的热点之一。本文提出了一种基于多种空间信息的高光谱遥感图像分类方法,将空间域和频域信息结合进行分类。实验结果表明,该方法具有较高的分类精度和准确性。关键词:高光谱遥感图像;分类方法;空间信息;频域信息引言高光谱遥感图像是通过采集高光谱数据并进行光谱分析得到的,具有很高的空间分辨率和光谱分辨率。高光谱遥感图像分类是对图像进行自动分类的过程,通过对图像中的像元进行
基于多特征图像集成的高光谱图像分类方法.docx
基于多特征图像集成的高光谱图像分类方法基于多特征图像集成的高光谱图像分类方法摘要:高光谱图像分类是遥感图像处理和分析的一项关键任务。针对高光谱图像中包含的大量光谱信息、空间信息以及上下文信息,本文提出了一种基于多特征图像集成的高光谱图像分类方法。该方法首先利用高光谱图像的光谱信息提取光谱特征,然后通过降维和选择算法进行特征选择,减少特征维度并提高分类性能。接着,利用高光谱图像的空间信息提取空间特征,采用纹理特征和形状特征进行空间特征提取和选择。最后,集成光谱特征和空间特征,通过分类器进行高光谱图像分类。实
基于多特征融合的遥感图像场景分类.docx
基于多特征融合的遥感图像场景分类基于多特征融合的遥感图像场景分类摘要:遥感图像场景分类在许多应用领域中起着重要的作用,如城市规划、环境管理和农业监测等。随着遥感技术的发展和传感器的多样化,遥感图像的特征也变得越来越丰富。然而,单一特征往往不能提供足够的信息来准确地进行场景分类。为了解决这个问题,本文提出了一种基于多特征融合的遥感图像场景分类方法。该方法将多种特征进行融合,以提高分类的准确性和鲁棒性。实验结果表明,本方法比传统的单一特征分类方法具有更好的性能。关键词:遥感图像,场景分类,多特征融合1.引言遥
基于SSAE深度学习特征表示的高光谱遥感图像分类方法.docx
基于SSAE深度学习特征表示的高光谱遥感图像分类方法摘要:高光谱遥感图像分类一直是遥感领域中的热门研究方向。为了提高分类准确率,本文提出了一种基于SSAE(StackedSparseAuto-Encoder)深度学习特征表示的高光谱遥感图像分类方法。在预处理阶段中,采用PCA(PrincipalComponentAnalysis)方法对高光谱遥感图像进行降维,再将降维后的数据输入到SSAE模型中进行训练,得到每个样本的深度学习特征表示。在分类阶段中,将特征映射到低维空间,采用SVM(SupportVect