
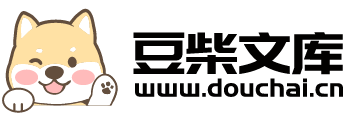
基于MapReduce的非线性支持向量机分类算法研究.docx
快乐****蜜蜂
在线预览结束,喜欢就下载吧,查找使用更方便
相关资料
基于MapReduce的非线性支持向量机分类算法研究.docx
基于MapReduce的非线性支持向量机分类算法研究Abstract:SupportVectorMachines(SVMs)havebeenwidelyusedinclassificationduetotheirexcellentclassificationperformance.However,traditionalSVMshavetheproblemofhighcomputationalcomplexitywhendealingwithalargeamountofdata.Tosolvethispro
基于MapReduce的非线性支持向量机分类算法研究的中期报告.docx
基于MapReduce的非线性支持向量机分类算法研究的中期报告一、研究背景随着互联网时代的到来,数据量变得越来越大,传统的机器学习算法已经无法满足数据建模和处理的需求。为了解决这个问题,Google提出了一种分布式计算框架MapReduce。这个框架的主要特点是可以将一个大规模的数据集分为许多小片段进行处理,因此可以大大提高计算效率和方便性。支持向量机(SVM)是一种常用的机器学习算法,它在处理二分类、多分类以及回归问题时都有很好的表现。但是,SVM对于大规模数据处理时存在一些问题,例如计算时间、内存消耗
基于MapReduce的非线性支持向量机分类算法研究的任务书.docx
基于MapReduce的非线性支持向量机分类算法研究的任务书一、研究背景和意义支持向量机(SupportVectorMachine,SVM)是机器学习中常用的分类算法之一,其在处理高维数据和非线性分类问题上具有很好的效果。然而,SVM在处理大规模数据集时,由于需要计算高维特征空间的内积和求解凸优化问题,计算时间和空间资源消耗较大,限制了算法的应用。为了解决这个问题,近年来出现了基于MapReduce的改进SVM算法,可以利用分布式计算和并行计算的优势,提高算法的效率和可扩展性。同时,非线性分类问题在现实生
基于Python的支持向量机分类算法研究.docx
摘要在大数据盛行的时代背景下,机器学习这门学科的广泛应用。并且列举运用Python语言进行数据处理的优势,将其与传统语言进行对比,充分体现了Python语言在语言简洁,效率高等方面的优势。这也是本文最后选择Python语言实现SVM算法的主要原因。本文主体内容阐述了支持向量机算法(SVM)的基本内涵,并且用图示和数学方法形象具体讲解了SVM的基本原理。具体分析了SVM算法中线性可分数据、线性不可分数据和含有outlier点的数据集的分类方式。通过对偶问题求解法、核函数、及SMO算法等实现了对最优超平面的求
基于Python的支持向量机分类算法研究.docx
摘要在大数据盛行的时代背景下,机器学习这门学科的广泛应用。并且列举运用Python语言进行数据处理的优势,将其与传统语言进行对比,充分体现了Python语言在语言简洁,效率高等方面的优势。这也是本文最后选择Python语言实现SVM算法的主要原因。本文主体内容阐述了支持向量机算法(SVM)的基本内涵,并且用图示和数学方法形象具体讲解了SVM的基本原理。具体分析了SVM算法中线性可分数据、线性不可分数据和含有outlier点的数据集的分类方式。通过对偶问题求解法、核函数、及SMO算法等实现了对最优超平面的求