
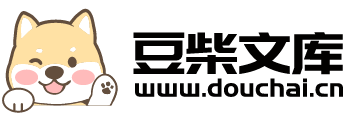
改进蚁群算法在TSP问题中的应用.docx
快乐****蜜蜂
在线预览结束,喜欢就下载吧,查找使用更方便
相关资料
改进蚁群算法在TSP问题中的应用.docx
改进蚁群算法在TSP问题中的应用Title:EnhancingAntColonyOptimizationforTSPProblemIntroduction:TheTravelingSalesmanProblem(TSP)isawell-knownoptimizationproblemincomputerscienceandoperationsresearch.Itinvolvesfindingtheshortestpossibleroutethatasalesmancantaketovisitasetof
蚁群算法在TSP问题中的应用.doc
单位代码01学号090111004分类号O24密级毕业论文蚁群算法在TSP问题中的应用院(系)名称信息工程学院专业名称信息与计算科学学生姓名王利超指导教师王爱苹2013年5月15日黄河科技学院毕业论文第页蚁群算法在TSP问题中的应用摘要蚁群算法是近年来发展起来的一种新型模拟进化算法,它是由意大利学者M.D0rigo等人在20世纪90年代初提出来的.这种算法模仿了蚂蚁在搬运食物的过程中,自发寻找最短路径的行为特征,加以改进并应用到不同的领域.蚁群算法作为一种新的
蚁群算法在TSP问题中的应用.doc
单位代码01学号090111004分类号O24密级毕业论文蚁群算法在TSP问题中的应用院(系)名称信息工程学院专业名称信息与计算科学学生姓名王利超指导教师王爱苹2013年5月15日黄河科技学院毕业论文第页蚁群算法在TSP问题中的应用摘要蚁群算法是近年来发展起来的一种新型模拟进化算法,它是由意大利学者M.D0rigo等人在20世纪90年代初提出来的.这种算法模仿了蚂蚁在搬运食物的过程中,自发寻找最短路径的行为特征,加以改进并应用到不同的领域.蚁群算法作为一种新的
蚁群算法在TSP问题中的应用.doc
单位代码01学号090111004分类号O24密级毕业论文蚁群算法在TSP问题中的应用院(系)名称信息工程学院专业名称信息与计算科学学生姓名王利超指导教师王爱苹2013年5月15日黄河科技学院毕业论文第页蚁群算法在TSP问题中的应用摘要蚁群算法是近年来发展起来的一种新型模拟进化算法,它是由意大利学者M.D0rigo等人在20世纪90年代初提出来的.这种算法模仿了蚂蚁在搬运食物的过程中,自发寻找最短路径的行为特征,加以改进并应用到不同的领域.蚁群算法作为一种新的
改进的蚁群算法在TSP中的应用.docx
改进的蚁群算法在TSP中的应用蚁群算法是一种模仿蚂蚁在寻找食物时行动的智能算法。它是一种基于群体智能的优化算法,可以应用于许多领域,包括旅行商问题(TSP)。本文将探讨改进的蚁群算法在TSP中的应用。旅行商问题(TSP)是一种NP难问题,涉及寻找一条路径以访问所有城市,并使路径的总长度最小。TSP是一个经典问题,因为它具有许多实际应用,例如物流、电子设计自动化和旅游规划。由于TSP的复杂性,许多算法被开发出来解决这个问题。其中蚁群算法就是一种。在传统的蚁群算法中,蚂蚁按照某种规则在城市之间移动,并在路径上