
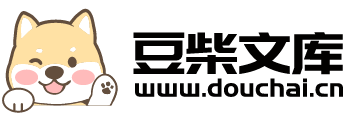
基于遗传算法的工厂AGV路径优化研究.docx
快乐****蜜蜂
在线预览结束,喜欢就下载吧,查找使用更方便
相关资料
基于遗传算法的工厂AGV路径优化研究.docx
基于遗传算法的工厂AGV路径优化研究AbstractFactoryAGV(AutomatedGuidedVehicle)systemisacrucialaspectofmodernsmartmanufacturing,whichfacilitatesefficientmaterialhandlingoperations.However,optimizingthepathofAGVsinafactorypresentsachallengingComputationalintelligenceproblem.
基于改进遗传算法的工厂AGV安全路径规划.docx
基于改进遗传算法的工厂AGV安全路径规划工厂AGV(自动导引车)的安全路径规划是一个重要且具有挑战性的课题,它直接关系到工厂生产效率和员工安全。由于工厂AGV在工厂生产过程中扮演了重要的角色,因此必须通过一定的算法来确保其能够在高效和安全的情况下运行。在本文中,将介绍一种基于改进遗传算法的工厂AGV安全路径规划算法。首先,我们首先需要确定工厂AGV的安全路径规划的目标和约束条件。该目标是为了使工厂AGV能够在最短的时间内到达目的地,同时保证车辆和周围环境的安全。对于约束条件,主要包括车辆最大速度、转向半径
基于改进遗传算法的AGV路径规划.docx
基于改进遗传算法的AGV路径规划基于改进遗传算法的AGV路径规划摘要AGV(AutomatedGuidedVehicle)路径规划在现代物流系统中起着重要作用。为了提高路径规划的效率和准确性,本文提出了一种基于改进遗传算法的AGV路径规划方法。首先,介绍了AGV的基本原理和路径规划的基本概念。然后,详细阐述了改进遗传算法的原理和步骤。接着,针对AGV路径规划的特点,提出了一种改进的适应度函数和交叉操作算子。最后,通过实验验证了改进后的遗传算法在AGV路径规划中的有效性和优越性。关键词:AGV,路径规划,遗
优化Dijkstra算法在工厂内物流AGV路径规划的研究.docx
优化Dijkstra算法在工厂内物流AGV路径规划的研究随着工业自动化和智能化的发展,工厂内物流AGV已经成为了现代制造业中不可缺少的一部分。而在工厂内,物流AGV的路径规划是至关重要的。传统的路径规划算法Dijkstra算法在工厂内物流AGV路径规划中表现较好,但存在一些问题。因此,本文将讨论关于如何优化Dijkstra算法在工厂内物流AGV路径规划中的应用。1.Dijkstra算法的优点Dijkstra算法属于单源最短路径算法,能够计算出源点到其他所有点的最短路径。在工厂内物流AGV路径规划中,起点和
基于遗传算法的路径优化问题研究.docx
基于遗传算法的路径优化问题研究基于遗传算法的路径优化问题研究摘要:路径优化问题是一个重要的研究领域,涉及到多个领域的应用。本文将探讨如何利用遗传算法来解决路径优化问题。首先,介绍了遗传算法的基本原理和步骤。然后,讨论了路径优化问题的定义和相关任务。接下来,详细分析了将遗传算法应用于路径优化问题的方法。最后,通过实例分析来验证算法的有效性和性能。关键词:路径优化问题,遗传算法,优化引言路径优化问题是指在给定的路径网络中,寻找最佳路径的问题。例如,在交通规划中,需要确定最佳的行驶路径以最大程度地减少时间和能源