
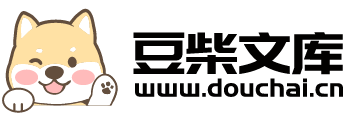
基于遗传算法优化BP神经网络的海上DFIG定子绕组故障诊断.docx
快乐****蜜蜂
在线预览结束,喜欢就下载吧,查找使用更方便
相关资料
基于遗传算法优化BP神经网络的海上DFIG定子绕组故障诊断.docx
基于遗传算法优化BP神经网络的海上DFIG定子绕组故障诊断摘要:近年来,海上风电发电机组作为一种可再生能源的重要形式,得到了广泛的应用和研究。然而,由于海上环境的复杂性,风电发电机组在运行过程中常常面临故障的挑战。其中,DFIG定子绕组故障是导致风电发电机组性能下降和损坏的主要原因之一。为了提高DFIG定子绕组故障的诊断准确性和效率,本文基于遗传算法优化BP神经网络,提出了一种海上DFIG定子绕组故障诊断方法。首先,本文针对DFIG定子绕组故障诊断问题,构建了适用于DFIG定子绕组故障特征提取的BP神经网
基于机电信号融合的DFIG定子绕组匝间短路故障诊断.docx
基于机电信号融合的DFIG定子绕组匝间短路故障诊断标题:基于机电信号融合的DFIG定子绕组匝间短路故障诊断摘要:随着可再生能源的快速发展,风力发电系统逐渐成为解决电力资源紧缺的重要手段。双馈异步发电机(DFIG)作为风力发电系统中常用的发电机类型,具有结构简单、转矩调节范围广、输出电压质量高等优点。然而,由于外界环境和使用条件的影响,DFIG定子绕组匝间短路故障的发生不能完全避免。因此,本论文基于机电信号融合的思想,研究了DFIG定子绕组匝间短路故障的诊断方法,以提高风力发电系统的可靠性和可维护性。第一章
基于改进遗传算法优化BP神经网络的变压器故障诊断.docx
基于改进遗传算法优化BP神经网络的变压器故障诊断标题:基于改进遗传算法优化BP神经网络的变压器故障诊断摘要:随着电力系统的快速发展,变压器作为电网中重要的能量转换设备,其状态的健康程度对电网的稳定运行具有重要影响。因此,对变压器的故障进行准确、高效的诊断显得尤为重要。本文基于改进遗传算法优化BP神经网络,针对变压器故障诊断问题进行了研究,通过优化神经网络的权值和阈值,提高了变压器故障诊断的准确性和性能。关键词:变压器故障诊断、改进遗传算法、BP神经网络、权值和阈值优化导言:随着电力系统的发展和电力设备的不
基于改进遗传算法优化BP神经网络的变压器故障诊断的综述报告.docx
基于改进遗传算法优化BP神经网络的变压器故障诊断的综述报告近年来,随着工业自动化和电力监测设备的广泛应用,变压器故障诊断成为电力系统中重要的研究方向。为了减少电力系统的停运时间和维护费用,需要开发可靠、快速、准确的变压器故障诊断方法。在此背景下,基于改进遗传算法的BP神经网络优化成为了一种新的变压器故障诊断方法。1.BP神经网络的基本原理BP神经网络是一种用于建立非线性函数的前馈神经网络。BP网络有效地克服了传统模型的限制,在分类、识别和预测方面取得了显著的成果。BP网络适用于处理包括复杂非线性关系的问题
基于遗传算法优化的BP神经网络的频谱预测方法.pdf
本发明公开了基于遗传算法优化的BP神经网络的频谱预测方法,涉及频谱预测技术领域,遗传算法GA作为进化算法的一种,可以模仿自然界生物体的遗传进化过程,是一种具有多次迭代过程的搜索算法,从而能够解决一系列最优化问题。本发明参照了自然界中生物体“适者生存,优胜劣汰”的自然选择生存法则,依据某些特定的适应度函数对种群中的各个个体进行遗传中的选择、突变和交叉并进行筛选,那些适应度较好的个体就会被选择以进行利用,而那些适应度较差的个体就被舍弃。经过遗传算法所留下来的个体既拥有了之前所存在的优势,还在此基础上有了更进一