
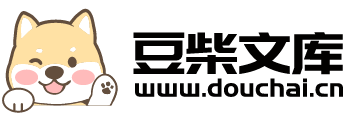
基于深度学习的LSTM光伏预测.docx
快乐****蜜蜂
在线预览结束,喜欢就下载吧,查找使用更方便
相关资料
基于深度学习的LSTM光伏预测.docx
基于深度学习的LSTM光伏预测Title:LSTM-basedDeepLearningforPhotovoltaicPowerGenerationPredictionAbstract:Asrenewableenergysourcesincreasinglycontributetoelectricitygeneration,accuratelypredictingthepoweroutputofphotovoltaic(PV)systemsbecomescrucialforoptimizingtheirin
基于MIE-LSTM的短期光伏功率预测.docx
基于MIE-LSTM的短期光伏功率预测基于MIE-LSTM的短期光伏功率预测摘要:随着太阳能光伏发电的快速发展,短期光伏功率预测对于控制和优化光伏系统的运行非常重要。本文提出了一种基于Multi-InputExcitationLSTM(MIE-LSTM)模型的短期光伏功率预测方法,该方法通过引入天气预报数据和历史功率数据来提高预测性能。实验结果表明,所提出的MIE-LSTM模型相比传统的LSTM模型具有更好的预测性能。1.引言随着全球能源需求的不断增长和对可再生能源的重视,太阳能光伏发电作为一种清洁、可持
基于EMD-LSTM的光伏发电预测模型.docx
基于EMD-LSTM的光伏发电预测模型基于EMD-LSTM的光伏发电预测模型摘要:随着可再生能源的发展,光伏发电作为一种清洁、可持续的能源形式被广泛应用。然而,光伏发电的波动性和不确定性给电力系统的运行和调度带来了挑战。因此,准确预测光伏发电功率的模型具有重要的意义。本文提出了一种基于经验模态分解(EmpiricalModeDecomposition,EMD)和长短期记忆神经网络(LongShort-TermMemory,LSTM)的光伏发电预测模型。关键词:光伏发电预测,经验模态分解,长短期记忆神经网络
基于LSTM的超短期光伏发电功率预测.docx
基于LSTM的超短期光伏发电功率预测标题:基于LSTM的超短期光伏发电功率预测摘要:随着光伏发电系统的普及和应用,准确预测光伏发电功率对于光伏电站的管理和运营至关重要。然而,光伏发电功率具有高度非线性、时变性和不确定性的特点,传统的预测方法往往难以满足精确预测的要求。本文基于长短期记忆网络(LSTM),通过对光伏发电功率数据进行训练,建立了一种准确的超短期光伏发电功率预测模型。实验结果表明,该模型能够有效地预测光伏发电功率的变化趋势,并具有较高的准确性。1.引言光伏发电作为清洁、可再生能源的代表,逐渐成为
基于FA-PCA-LSTM的光伏发电短期功率预测.docx
基于FA-PCA-LSTM的光伏发电短期功率预测基于FA-PCA-LSTM的光伏发电短期功率预测摘要:光伏发电是一种重要的可再生能源形式,对于实现清洁能源的目标具有重要意义。然而,由于日光和气象等因素的影响,光伏发电的短期功率预测面临着巨大的挑战。本文提出了一种基于因子分析(FactorAnalysis)、主成分分析(PrincipalComponentAnalysis)和长短期记忆网络(LongShort-TermMemory,LSTM)的光伏发电短期功率预测方法,称为FA-PCA-LSTM。该方法首先