
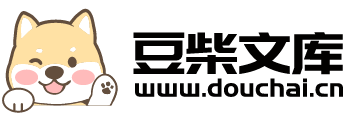
基于多图谱的脑MRI海马体分割方法研究.docx
快乐****蜜蜂
在线预览结束,喜欢就下载吧,查找使用更方便
相关资料
基于多图谱的脑MRI海马体分割方法研究.docx
基于多图谱的脑MRI海马体分割方法研究基于多图谱的脑MRI海马体分割方法研究摘要:脑MRI海马体分割是神经影像学领域中的一个重要任务,对于研究和诊断神经退行性疾病具有重要的意义。然而,由于脑MRI图像存在噪声、纹理不清晰等问题,传统的基于单个图谱的分割方法在海马体分割中存在一定的局限性。本文针对该问题,提出了一种基于多图谱的脑MRI海马体分割方法。第一部分:介绍1.引言:介绍海马体的重要性,以及脑MRI海马体分割的意义和现状问题。2.相关工作:回顾了海马体分割的现有方法,指出了其局限性和不足之处。第二部分
基于多图谱的脑MRI海马体分割方法研究的任务书.docx
基于多图谱的脑MRI海马体分割方法研究的任务书一、研究背景MRI技术已经成为现代医疗图像领域的重要手段之一,能够对人体组织进行非侵入式的成像,并且在医疗、医学研究等领域得到了广泛的应用。其中,MRI海马体分割是一项关键技术,因为海马体在脑部相关疾病的诊断、预测和研究中起着至关重要的作用。传统的MRI海马体分割方法通常基于手工制作的特征提取和分类器,存在着分割精度低、灵活性差等问题。针对这些问题,基于多图谱的MRI海马体分割方法成为了研究的热点之一。二、研究目的本研究旨在探究基于多图谱的MRI海马体分割方法
基于多图谱配准的海马体自动分割方法研究.docx
基于多图谱配准的海马体自动分割方法研究随着医学影像技术的逐渐发展,人们已经能够准确地对人体内部的各种器官结构进行观察和分析。在诸如神经科学、心血管学、肿瘤学等领域中,图像分割技术已经成为了一项非常重要和基础的技术。然而,在进行图像分割的过程中,由于图像边缘不清和噪声等因素,其精度和效率受到了很大的影响。本文旨在探讨基于多图谱配准的海马体自动分割方法的研究。海马体是大脑中一个非常重要的结构,并且在研究中扮演着重要的角色。因此,准确地对其进行分割是非常必要的。基于多图谱配准的海马体自动分割方法的研究,主要是针
基于多图谱标签融合的脑MRI图像分割.docx
基于多图谱标签融合的脑MRI图像分割摘要本论文提出了一种基于多图谱标签融合的脑MRI图像分割方法。该方法首先对脑MRI图像进行预处理,并利用多个标准脑图谱作为参考,进行多个图谱标签融合得到最终的分割结果。与单个图谱方法相比,该方法在精度和鲁棒性上都有所提高。实验证明,该方法能够有效地进行脑MRI图像分割,可为临床医学提供帮助。关键词:脑MRI图像分割、多图谱标签融合、标准脑图谱、精度、鲁棒性AbstractThispaperproposesabrainMRIimagesegmentationmethodb
基于多图谱配准的海马体自动分割方法研究的中期报告.docx
基于多图谱配准的海马体自动分割方法研究的中期报告摘要:本文研究了基于多图谱配准的海马体自动分割方法。该方法可以自动地对海马体进行分割,并且能够很好地适应不同分辨率和不同扫描方式的数据。在本文中,我们介绍了该方法的原理和实现,并且对其进行了初步的验证。介绍:海马体是大脑中一个非常重要的结构,它与记忆、空间定位等多个认知功能有着密切的联系。因此,对海马体进行研究是当前神经科学领域的一个热点问题。然而,海马体的手动分割非常耗时且容易受到人为因素的影响,因此自动分割方法变得越来越重要。近年来,基于多图谱配准的自动