
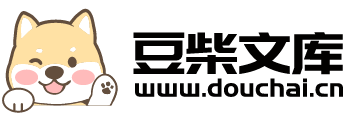
结合分层和ADMM的高光谱图像解混方法.docx
快乐****蜜蜂
在线预览结束,喜欢就下载吧,查找使用更方便
相关资料
结合分层和ADMM的高光谱图像解混方法.docx
结合分层和ADMM的高光谱图像解混方法Title:AHybridHyperspectralImageUnmixingMethodusingLayeredandADMMApproachesAbstract:Hyperspectralimageunmixingisafundamentaltaskinremotesensing,aimingtoestimatetheconstituentsandtheirabundancesinmixedpixelspectra.Thispaperpresentsanovela
高精度船舶高光谱图像的解混方法.pptx
高精度船舶高光谱图像的解混方法目录添加章节标题高精度船舶高光谱图像解混方法概述解混方法的重要性和应用场景解混方法的分类和比较解混方法的未来发展趋势高精度船舶高光谱图像解混方法的基本原理图像解混的基本概念和原理图像解混的数学模型和算法流程图像解混的关键技术问题和解决方案高精度船舶高光谱图像解混方法的具体实现图像预处理和数据准备特征提取和选择分类器的选择和训练解混结果的评价和优化高精度船舶高光谱图像解混方法的实际应用案例案例一:某海域船舶检测与识别案例二:某港口船舶分类与统计案例三:某河流船舶污染源追溯案例四
高光谱遥感图像光谱解混方法研究及其应用.docx
高光谱遥感图像光谱解混方法研究及其应用高光谱遥感图像光谱解混方法研究及其应用摘要:高光谱遥感图像是近年来遥感技术发展的重要成果之一,其具有丰富的光谱信息,可以提供更多的地物识别和分类的能力。然而,高光谱遥感图像中存在的混合像素问题限制了其在地物提取和应用中的准确性。因此,本研究旨在探讨高光谱遥感图像中的光谱解混方法,并通过实验证明其在不同应用场景下的有效性。1.引言高光谱遥感图像是多光谱遥感图像的发展成果,其具有比传统遥感图像更高的光谱分辨率。高光谱遥感图像可以提供丰富的光谱信息,使得在地物识别和分类中具
基于光谱和空间信息的高光谱图像解混方法研究的开题报告.docx
基于光谱和空间信息的高光谱图像解混方法研究的开题报告一、研究背景:高光谱技术是一种非常有前景的技术,它能够获取更加全面、详细的光谱信息,从而更加深入地理解物体的属性和结构。由于物体较为复杂,导致解混任务成为高光谱图像处理的瓶颈。因此,高光谱图像的解混过程成为高光谱图像处理中的重要研究内容。二、研究意义:高光谱图像解混问题是高光谱图像处理中的重要挑战,在农业、环境、医学等众多领域有着重要的应用。如在农业领域中,通过对农作物的高光谱图像进行解混,可以更加全面、准确地评估作物生长状况,为农业生产提供决策依据。同
高光谱图像的分类与解混研究.docx
高光谱图像的分类与解混研究高光谱图像的分类与解混研究摘要:本论文主要介绍高光谱图像的分类与解混研究。高光谱图像是一种可以获取大量光谱信息的图像,具有多光谱特征,可以广泛应用于农业、环境、地质等领域。然而,高光谱图像中存在大量的冗余信息和高噪声,导致分类和解混的困难。因此,本文主要从特征提取、分类算法和解混算法等方面对高光谱图像的分类与解混进行研究。1.引言高光谱图像是通过采集物体在可见光和近红外光谱范围内的反射和辐射能量所形成的图像。相对于传统的彩色图像,高光谱图像具有更多的光谱信息,可以提供更多的细节和