
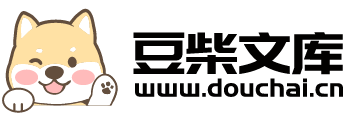
基于改进粒子群算法的PIDNN解耦控制研究(英文).docx
快乐****蜜蜂
在线预览结束,喜欢就下载吧,查找使用更方便
相关资料
基于改进粒子群算法的PIDNN解耦控制研究(英文).pptx
基于改进粒子群算法的PIDNN解耦控制研究目录添加章节标题引言研究背景和意义国内外研究现状研究目的和内容改进粒子群算法粒子群算法简介改进粒子群算法的提出改进粒子群算法的实现改进粒子群算法的性能评估PIDNN模型构建PIDNN模型简介PIDNN模型的基本结构PIDNN模型的参数确定PIDNN模型的学习和训练基于改进粒子群算法的PIDNN解耦控制方法解耦控制简介基于改进粒子群算法的PIDNN解耦控制方法的提出基于改进粒子群算法的PIDNN解耦控制方法的实现基于改进粒子群算法的PIDNN解耦控制方法的性能评估实
基于改进粒子群算法的PIDNN解耦控制研究(英文).docx
基于改进粒子群算法的PIDNN解耦控制研究(英文)ResearchonImprovedParticleSwarmAlgorithm-basedPIDNNDecouplingControlAbstract:Inrecentyears,therehasbeenanincreasinginterestinthedevelopmentofintelligentcontrolalgorithmsforcomplexsystems.Thispaperproposesanimprovedparticleswarmalg
基于粒子群算法优化PIDNN的温室系统解耦控制.docx
基于粒子群算法优化PIDNN的温室系统解耦控制基于粒子群算法优化PIDNN的温室系统解耦控制摘要:温室系统作为一种智能化的农业生产方式,对于植物的种植和生长环境进行精确的调控和控制。而温室系统中的温度和湿度这两个重要的参数互相影响,因此需要进行解耦控制,以实现更好的温室环境控制。本论文基于粒子群算法优化的PID神经网络(PIDNN)来实现温室系统的解耦控制,模拟和分析了PIDNN在温室系统中的应用效果。关键词:温室系统,解耦控制,粒子群算法,PIDNN1.引言温室系统是一种对植物生长环境进行精确控制的方法
基于改进粒子群算法的活套系统解耦控制研究(英文).docx
基于改进粒子群算法的活套系统解耦控制研究(英文)Title:ResearchonDecouplingControlofActiveStructuresBasedonImprovedParticleSwarmOptimizationAbstract:Inrecentyears,thecontrolofactivestructureshasbecomeanimportantresearchfieldduetoitspotentialapplicationsinvariousengineeringdiscipl
基于粒子群算法的PID神经网络解耦控制.docx
基于粒子群算法的PID神经网络解耦控制基于粒子群算法的PID神经网络解耦控制摘要:随着工业自动化的快速发展,控制系统在实际应用中面临着越来越多的挑战。这些挑战主要来自于工业过程中的非线性、不确定性以及耦合效应等因素。为了提高控制系统的性能,本论文提出了一种基于粒子群算法的PID神经网络解耦控制方法。该方法使用PID控制算法和神经网络技术相结合,通过采用粒子群算法调节神经网络的权重和偏置参数,实现对多变量耦合系统的解耦控制。实验结果表明,该方法在解耦效果、稳定性和鲁棒性等方面均取得了良好的控制效果,有效提高