
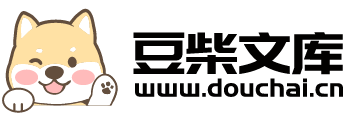
基于改进混沌搜索的AMPSO-BP短期负荷预测.docx
快乐****蜜蜂
在线预览结束,喜欢就下载吧,查找使用更方便
相关资料
基于改进混沌搜索的AMPSO-BP短期负荷预测.docx
基于改进混沌搜索的AMPSO-BP短期负荷预测Title:ImprovedChaoticSearchBasedAMPSO-BPShort-termLoadForecastingAbstract:Short-termloadforecastingplaysacrucialroleintheeffectiveoperationofpowersystems.Accuratepredictionsoffutureelectricitydemandhelpensuregridstabilityandoptimize
基于混沌神经网络的电力负荷短期预测.docx
基于混沌神经网络的电力负荷短期预测基于混沌神经网络的电力负荷短期预测摘要:电力负荷的短期预测对于电力系统的可靠运行和优化调度有着重要的意义。本文提出了一种基于混沌神经网络的电力负荷短期预测方法。首先,利用混沌序列产生器生成的混沌序列作为输入数据的处理,使得输入数据具有更高的随机性和不可预测性。然后,引入神经网络模型进行负荷预测,通过训练网络模型来学习负荷数据的变化规律。最后,通过对比实际负荷数据和预测结果,验证了该方法的有效性。关键词:电力负荷;短期预测;混沌序列;神经网络引言:电力负荷预测是电力系统运行
基于改进的SVM短期负荷预测研究.docx
基于改进的SVM短期负荷预测研究随着电力系统规模的不断扩大和负荷需求的增加,如何准确预测电力负荷就成为了电力生产和供应管理中的一个重要问题。准确的负荷预测可以帮助电力企业进行有效调度和计划,提高电力系统的稳定性和安全性,减少电力损失和成本。因此,负荷预测在电力系统运行和管理中扮演重要的角色。传统的负荷预测方法采用线性模型,如ARIMA模型和回归分析,在一定程度上可以进行负荷预测,但随着电力系统复杂度和不确定性的增加,线性模型已经无法满足负荷预测的需求。因此,使用机器学习方法来进行负荷预测成为了趋势。支持向
基于混沌理论及小波理论的短期负荷预测.pdf
第33卷第1期四川电力技术Vo.l33,No.12010年2月SichuanElectricPowerTechnologyFeb.,2010基于混沌理论及小波理论的短期负荷预测李凌舟1,赵华阳2,席 川1(1.四川电力职业技术学院,四川成都 610072;2.中电投电力工程有限公司海阳核电项目部,山东海阳 265116)摘 要:分析了多种负荷预测的方法,着重分析了负荷的混沌特性与小波特性,同时分析了小波变换能够反应负荷的变化趋势与随机因素。利用Matlab工具,建立了基于小波理论与混沌理论相结合的负荷预测
基于混沌时间序列法的微网短期负荷预测.docx
基于混沌时间序列法的微网短期负荷预测随着可再生能源技术的发展以及加强对能源可持续性的需求,微网得到了广泛的关注和研究。在微网运行过程中,对负荷的准确预测是十分重要的。本文将对基于混沌时间序列方法的微网短期负荷预测方法进行探讨和分析。一、短期负荷预测的意义微网中负荷的变化与能源供给不断变化的关系密切相关,因此短期负荷预测是保证微网稳定运行的必要条件之一。正确预测短期负荷可以帮助微网的管理者制定出合理的运行计划,避免能源浪费和缺乏能源的情况的发生。二、混沌时间序列方法简介混沌时间序列方法是一种对时间序列进行预