
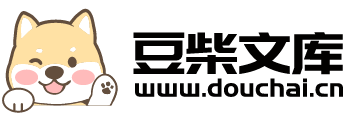
基于特征约简的随机森林改进算法研究.docx
快乐****蜜蜂
在线预览结束,喜欢就下载吧,查找使用更方便
相关资料
基于特征约简的随机森林改进算法研究.docx
基于特征约简的随机森林改进算法研究基于特征约简的随机森林改进算法研究摘要随机森林是一种训练高度准确的分类和回归模型的集成学习算法。然而,对于高维数据集,随机森林往往会遇到问题,如特征冗余和过拟合。为了解决这些问题,本文提出了一种基于特征约简的随机森林改进算法。本文首先介绍了随机森林的基本原理和特点,然后讨论了高维数据集中存在的问题,并分析了特征约简的重要性。接着,本文详细描述了提出的随机森林改进算法。该算法的核心思想是通过特征约简来减少冗余特征,并提高模型的泛化能力。具体而言,算法首先使用随机森林生成初始
基于特征选择的极限随机森林算法研究.docx
基于特征选择的极限随机森林算法研究引言:在机器学习领域中,特征选择是极其重要的一个领域,它可以提取出最具代表性的特征,以此预测模型的精度和准确度。然而,在实践中,特征选择面临着许多复杂问题,例如高维数据、冗余、缺失或无用的数据以及不同范围和分布的特征等等,这些问题都需要解决以确保特征选择的正确性和有效性。针对这一问题,目前有很多特征选择算法被提出和发展,其中极限随机森林算法是近年来非常有潜力的一种算法,本文旨在对其进行研究和分析。正文:一、特征选择的意义和发展历程特征选择是机器学习领域的一个重要环节,特征
基于特征选择的极限随机森林算法研究.docx
基于特征选择的极限随机森林算法研究摘要随着大数据时代的到来,随机森林算法成为了一个重要的机器学习工具。它具有良好的准确性和鲁棒性,已经在许多领域得到了广泛应用。然而,对于高维数据,随机森林算法的表现有时候不尽人意,可能会遇到过拟合和膨胀等问题。为了解决这些问题,我们提出了基于特征选择的极限随机森林算法。该算法采用基于方差的特征选择方法对数据进行预处理,从而剔除一些与目标变量无关的冗余特征。然后,使用剩余特征构建随机森林。最后,采用集成投票的方式对原数据以及剩余特征构建的随机森林生成的分类结果进行整合,从而
基于森林优化特征选择算法的改进研究.docx
基于森林优化特征选择算法的改进研究基于森林优化特征选择算法的改进研究摘要:特征选择在机器学习和数据挖掘中起着至关重要的作用。因为过多或不相关的特征会增加计算复杂度,降低模型的解释性和预测性能。森林优化特征选择算法(OFSA)是一种基于优化算法的特征选择方法,它模拟物种进化的过程,通过优化自适应权重实现特征选择。然而,OFSA在大规模数据集上存在计算复杂度高的问题和容易陷入局部最优问题。本文通过将OFSA与遗传算法和粒子群优化算法相结合,提出了一种改进的森林优化特征选择算法(IOFSA)。实验结果表明,IO
基于改进随机森林算法的多规格货物装载研究.docx
基于改进随机森林算法的多规格货物装载研究标题:基于改进随机森林算法的多规格货物装载研究摘要:多规格货物装载问题是一个重要的组合优化问题,涉及到物流、运输和仓储等领域。本论文基于改进的随机森林算法,对多规格货物装载问题进行研究。首先,我们介绍了多规格货物装载问题的背景和意义。然后,基于随机森林算法的原理和优缺点进行了详细的分析。接下来,我们提出了改进的随机森林算法,并针对多规格货物装载问题进行了具体的应用实验。实验结果表明,改进的随机森林算法在解决多规格货物装载问题上具有较好的性能表现。关键词:多规格货物装