
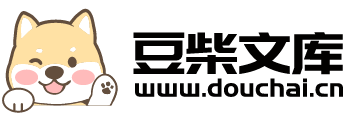
基于卷积-LSTM网络的广告点击率预测模型研究.docx
快乐****蜜蜂
在线预览结束,喜欢就下载吧,查找使用更方便
相关资料
基于卷积-LSTM网络的广告点击率预测模型研究.docx
基于卷积-LSTM网络的广告点击率预测模型研究Title:ResearchonConvolutional-LSTMBasedModelforClick-ThroughRatePredictioninAdvertisingAbstract:Withtherapidgrowthofonlineadvertising,accuratelypredictingtheclick-throughrate(CTR)hasbecomecrucialforadvertiserstooptimizetheirmarketin
基于FTRL优化算法的广告点击率预测模型研究.pptx
添加副标题目录PART01FTRL算法的基本原理FTRL算法的优势和特点FTRL算法的应用场景PART02广告点击率预测的重要性广告点击率预测模型的构建广告点击率预测模型的评估指标PART03FTRL在广告点击率预测中的应用基于FTRL的广告点击率预测模型的实现基于FTRL的广告点击率预测模型的优势和局限性PART04数据集的选择与预处理实验设置与参数调整实验结果与分析与其他算法的比较PART05基于FTRL的广告点击率预测模型的优化方向FTRL算法在其他领域的拓展应用未来研究展望感谢您的观看
基于Stacking模型集成的LSTM网络短期负荷预测研究.docx
基于Stacking模型集成的LSTM网络短期负荷预测研究基于Stacking模型集成的LSTM网络短期负荷预测研究摘要:LSTM网络是一种广泛应用于时间序列预测领域的深度学习模型,它具有处理长短期依赖关系的优势。然而,单独使用LSTM网络进行负荷预测面临一些限制,如过拟合和预测性能不稳定。为了解决这些问题,本研究提出了一种基于Stacking模型集成的LSTM网络负荷预测方法。该方法首先利用多种特征进行数据预处理,然后将其输入到多个LSTM网络中进行训练,最后通过Stacking模型进行集成预测。实验证
基于深度网络模型压缩的广告点击率预估模型.pptx
汇报人:目录PARTONEPARTTWO模型剪枝技术知识蒸馏技术量化技术混合精度训练技术PARTTHREE广告点击率预估的挑战深度学习在广告点击率预估中的应用基于深度网络模型压缩的广告点击率预估模型的优势广告点击率预估模型的评估指标PARTFOUR剪枝技术在广告点击率预估中的应用知识蒸馏技术在广告点击率预估中的应用量化技术在广告点击率预估中的应用混合精度训练技术在广告点击率预估中的应用PARTFIVE实验数据集与实验环境实验方法与实验过程实验结果与分析结果对比与讨论PARTSIX基于深度网络模型压缩的广告
基于用户画像的互联网广告点击率预测模型研究.pptx
汇报人:/目录0102用户画像的定义用户画像的构成要素用户画像的获取方法03广告点击率预测模型的定义广告点击率预测模型的原理广告点击率预测模型的构建过程04用户画像与广告点击率的关系基于用户画像的广告点击率预测模型的优势基于用户画像的广告点击率预测模型的实现方法05实验数据集的选择与处理实验方法与实验过程实验结果分析结果对比与讨论06基于用户画像的广告点击率预测模型的优化方向未来研究展望汇报人: