
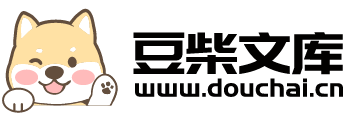
改进粒子群算法优化SVR水质预测模型研究.docx
骑着****猪猪
在线预览结束,喜欢就下载吧,查找使用更方便
相关资料
改进粒子群算法优化SVR水质预测模型研究.docx
改进粒子群算法优化SVR水质预测模型研究AbstractInrecentyears,supportvectorregression(SVR)hasgainedwidepopularityinwaterqualityprediction,owingtoitspowerfullearningabilityandhighpredictionaccuracy.However,theconventionalSVRmodeloftengetstrappedinlocaloptimizationandfailstofi
基于趋化-改进粒子群算法的SVR光合速率预测模型.docx
基于趋化-改进粒子群算法的SVR光合速率预测模型基于趋化-改进粒子群算法的SVR光合速率预测模型摘要:光合速率是指植物在光照条件下进行光合作用的速率,是植物生长和光合效率的重要指标。准确预测光合速率对于农业生产和环境研究具有重要意义。本论文针对光合速率预测问题,根据支持向量回归(SVR)模型,提出了一种基于趋化-改进粒子群算法的光合速率预测模型。该模型通过引入趋化操作和改进粒子群算法,提高了SVR模型的预测精度和训练速度。实验结果表明,该模型在光合速率预测方面具有较好的表现。关键词:光合速率、SVR、趋化
基于改进粒子群优化算法的BP预测模型.docx
基于改进粒子群优化算法的BP预测模型摘要:BP神经网络在数据预测和分类问题中有着广泛应用,但其训练时间长、易过拟合等问题也不容忽视。为此,本文提出了一种基于改进粒子群优化算法的BP预测模型,通过优化BP算法的权重和阈值,使得神经网络达到更好的预测效果。通过实验验证,本文模型相比传统BP算法,具有更快的训练时间和更优的预测精度。关键词:BP神经网络,粒子群优化算法,预测模型,过拟合1.引言数据挖掘和机器学习算法在数据预测和分类问题中有着广泛应用。近年来,人工神经网络(ANN)已成为了诸多研究领域中的最佳算法
改进粒子群算法优化的非线性模型预测控制.docx
改进粒子群算法优化的非线性模型预测控制随着工业化和自动化的发展,非线性系统控制问题变得越来越重要,如何提高控制系统的运行性能一直是控制领域研究的重点。其中,预测控制由于其对未来控制信号的预测和反馈纠正,被广泛应用于非线性系统控制中。而粒子群算法由于其高效性和全局搜索能力已成为常用的优化算法之一。在本文中,我们主要关注如何改进粒子群算法优化的非线性模型预测控制。一、粒子群算法的原理粒子群算法是一种模拟物种社会行为的仿生算法,由Eberhart和Kenned在1995年提出。其基本思想是通过模拟物种的飞行和寻
粒子群优化算法的改进研究.docx
粒子群优化算法的改进研究1.引言粒子群优化算法(ParticleSwarmOptimization,PSO)是由Kennedy和Eberhart于1995年提出的基于群体智能的全局优化算法。PSO算法具有简单易实现、收敛速度快等优点,在许多实际问题中得到了广泛应用。但随着应用场景复杂度增加,PSO算法不足逐渐显现。因此,本文针对PSO算法的不足,主要探讨了多个改进方案并进行了对比实验和分析。2.PSO算法的基本思想PSO算法基于一个群体的行为,在一个连续的空间中通过一组粒子模拟优化过程。每个粒子的位置代表