
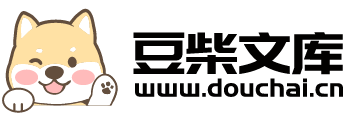
基于用户动态兴趣的微博个性化推荐的研究.docx
快乐****蜜蜂
在线预览结束,喜欢就下载吧,查找使用更方便
相关资料
基于用户动态兴趣的微博个性化推荐的研究.docx
基于用户动态兴趣的微博个性化推荐的研究基于用户动态兴趣的微博个性化推荐的研究AbstractWiththewidespreadusageofsocialmediaplatformssuchasWeibo,personalizedrecommendationshavebecomeincreasinglyimportantforenhancinguserexperiences.Inthispaper,weproposearesearchframeworkforpersonalizedWeiborecommen
基于用户动态兴趣的微博个性化推荐的研究的中期报告.docx
基于用户动态兴趣的微博个性化推荐的研究的中期报告一、研究背景随着社交网络的兴起,微博平台成为越来越多人交流、获取信息的首选。然而,微博中蕴含的信息量大,涉及范围广,用户需要花费大量时间才能找到自己感兴趣的内容。针对这一问题,以用户动态兴趣为基础的微博个性化推荐成为了研究热点。本次研究旨在基于用户历史行为和互动模式构建用户兴趣模型,进一步提高微博推荐的准确性和个性化程度。二、研究目标本次研究的目标是构建一个基于用户行为与兴趣关系的微博个性化推荐模型,从而提升微博推荐的准确性和用户体验。具体目标如下:1.建立
基于微博用户兴趣的个性化信息推荐方法研究.docx
基于微博用户兴趣的个性化信息推荐方法研究摘要:随着微博用户数量的不断增加,如何从海量的微博信息中为用户提供个性化的推荐服务成为了一个热门的研究领域。本文探讨了基于微博用户兴趣的个性化信息推荐方法,主要涉及用户兴趣模型构建、内容相似度计算以及推荐算法等方面。通过实验验证,本文提出的推荐方法能够显著提高用户的推荐准确率和效果。关键词:微博用户,个性化推荐,兴趣模型,内容相似度,推荐算法Introduction微博是一种新兴的社交网络平台,它为用户提供了一种便捷的方式来分享自己的生活、工作和兴趣爱好。在微博上,
基于微博用户兴趣的个性化信息推荐方法研究的中期报告.docx
基于微博用户兴趣的个性化信息推荐方法研究的中期报告一、研究背景和意义随着社交媒体的发展,微博平台已经成为了人们分享信息、获取信息、交流思想的重要渠道之一。然而,由于每个用户的兴趣爱好各不相同,不同用户所关注的信息也有所不同,因此对于每个用户来说,如何在众多的微博信息中快速找到自己感兴趣的内容就成了一个十分重要的问题。而个性化信息推荐技术的出现,很好地解决了这一问题,可以为每个用户提供更符合其兴趣爱好的信息,加强用户与平台的互动,提高平台的用户满意度。因此,基于微博用户兴趣的个性化信息推荐方法研究具有重要的
基于用户建模的个性化微博推荐研究.docx
基于用户建模的个性化微博推荐研究摘要随着互联网的发展,微博作为一种新兴的社交媒体平台,已成为人们获取信息和娱乐的重要渠道。然而,随着微博用户数量的增加,用户每天面对的微博数量也在不断增加,从而产生了信息过载的问题,这给用户的阅读和筛选带来了很大的困难。因此,推荐系统作为一种解决这一问题的有效方法,受到了越来越多的关注。本文提出了一种基于用户建模的个性化微博推荐算法,主要包括用户建模、微博表示和推荐模型三个部分。实验结果表明,与传统推荐算法相比,该算法可以更准确和有效地推荐用户感兴趣的微博内容,为用户提供更