
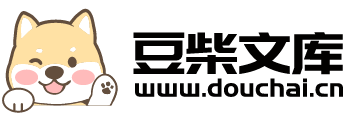
基于参数化模型的遥感图像匀光算法.docx
快乐****蜜蜂
在线预览结束,喜欢就下载吧,查找使用更方便
相关资料
基于参数化模型的遥感图像匀光算法.docx
基于参数化模型的遥感图像匀光算法Abstract:Imageuniformizationisanimportantpreprocessingstepinmanyremotesensingapplications.Traditionalmethodsoftenrelyonhandcraftedfeaturesorintensecomputation,whichlimitstheirefficiencyandpracticality.Parameterizedmodelsprovideanewsolution
基于参数化模型的遥感图像匀光算法的开题报告.docx
基于参数化模型的遥感图像匀光算法的开题报告一、研究背景和研究意义随着遥感技术的不断发展和普及,遥感图像已经广泛应用于地质勘探、资源调查、城市规划、农业生产等领域。不过,由于环境、天气等各种因素的影响,遥感图像中常常存在光照不均匀的问题,即图像的某些区域比其他区域亮度更高或更低,这会影响图像的质量和后续处理结果的准确性。因此,如何解决遥感图像的光照不均匀问题,是遥感图像处理研究的重要课题之一。目前,针对遥感图像光照不均匀问题,已经有许多方法被提出。其中比较典型的方法是基于传统的统计学方法,如中值滤波、均值滤
基于IDL的遥感影像匀光算法研究与实现的开题报告.docx
基于IDL的遥感影像匀光算法研究与实现的开题报告一、研究的背景和意义近年来,遥感技术在国家经济建设和农业生产方面的应用越来越广泛。然而,由于种种原因,获取的遥感图像往往存在着光照不均匀的问题,即某些地区的亮度过高,而另一些地区则过低,这一问题严重影响了图像的正常应用。因此,遥感图像的匀光处理成为遥感图像处理中的重要环节之一。对于遥感图像匀光处理,目前已有各种方法,如直方图匹配、局部对比度增强、频域滤波法等。然而,这些方法在不同图像场景下的效果十分不尽相同。因此,为了更有效地实现遥感图像匀光处理,本研究采用
基于IDL的遥感影像匀光算法研究与实现的任务书.docx
基于IDL的遥感影像匀光算法研究与实现的任务书任务书任务名称:基于IDL的遥感影像匀光算法研究与实现任务目的:随着卫星成像技术不断的发展,获取高分辨率遥感影像数据已成为了遥感学科及其应用领域的核心。但是,由于大气、云、地理环境、地形和地物的影响,遥感影像数据中往往会出现图像的不均匀性,即基于光学遥感影像相同地物的亮度和颜色会因相机光学系统和大气扰动等因素的影响而出现较大的变化,这就需要进行遥感影像的匀光操作以进一步提高遥感影像数据的质量和可用性。因此,本次任务旨在研究和实现一种基于IDL的遥感影像匀光算法
基于地面参数的遥感图像并行仿真算法研究的中期报告.docx
基于地面参数的遥感图像并行仿真算法研究的中期报告一、研究背景随着遥感技术的不断发展,遥感图像处理和分析已成为了地理信息系统、城市规划、资源管理、环境保护等领域的基础。在遥感图像处理和分析中,地面参数的估算是一个重要的研究方向。通过估算地面参数,可以更好地理解和解释遥感图像的信息,为相关领域的决策提供科学依据。有许多方法可以用于估算地面参数,如植被指数、土地利用/覆盖分类等。然而,这些方法大多需要大量的计算和处理,因此需要高效的算法和技术支持。并行计算是一种重要的方法,可以显著提高数据处理和计算速度,使得地