
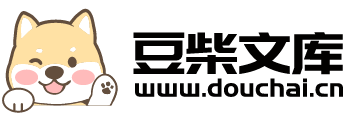
基于Faster R-CNN的隧道图像裂缝检测.docx
骑着****猪猪
在线预览结束,喜欢就下载吧,查找使用更方便
相关资料
基于Faster R-CNN的隧道图像裂缝检测.docx
基于FasterR-CNN的隧道图像裂缝检测摘要特别是在隧道工程中,裂缝的形成会影响结构的稳定性和安全性,因此裂缝检测是很重要的工作。传统的裂缝检测方法通常需要耗费大量的时间和人力,而且准确率低。本研究基于FasterR-CNN模型提出了一种新的隧道图像裂缝检测算法,该算法可以有效地提高检测速度和准确率。首先,针对传统的裂缝检测方法存在的问题,本研究提出了利用FasterR-CNN模型进行裂缝检测的思路。然后,我们对FasterR-CNN模型进行了修改,优化了模型中的特征提取器,增加了多任务学习和损失函数
基于Faster Rcnn的图像文本检测方法及系统.pdf
本发明涉及图像处理技术领域,公开了一种基于FasterRcnn的图像文本检测方法及系统,其方法通过对待检测图像进行预处理,以提高图像的成像质量,并通过目标检测算法对文本信息进行定位,通过定位信息利用OCR识别算法对文本信息进行文本识别,将文本信息转换为字符信息,从而提高图像识别的准确性。
基于改进Faster RCNN与Grabcut的商品图像检测.docx
基于改进FasterRCNN与Grabcut的商品图像检测商品图像检测一直是计算机视觉领域的研究热点之一。在基于深度学习的方法中,FasterRCNN是一种非常经典和有效的目标检测算法,而Grabcut则是一种常用的图像分割算法。本文将基于改进的FasterRCNN和Grabcut算法提出一种商品图像检测方法,以提高商品检测的准确性和效率。首先,我们简要介绍一下FasterRCNN算法的基本原理。FasterRCNN是一种两阶段目标检测算法,它由两个主要组件组成:RegionProposalNetwork
基于Faster-RCNN的遥感图像飞机检测算法.docx
基于Faster-RCNN的遥感图像飞机检测算法基于Faster-RCNN的遥感图像飞机检测算法摘要:随着遥感技术的发展,遥感图像在各个领域得到了广泛应用。其中,遥感图像中的目标检测一直是研究的热点,而飞机检测作为其中的一个重要任务也备受关注。本文提出了基于Faster-RCNN的遥感图像飞机检测算法,并对其进行了详细的研究和评估。实验结果表明,该算法在飞机检测任务上具有较高的性能和鲁棒性。1.引言随着航空技术的快速发展,遥感图像已经成为获取大范围地表信息的重要手段。遥感图像中的目标检测一直是研究的热点,
基于Faster RCNN的图像配准算法研究.docx
基于FasterRCNN的图像配准算法研究基于FasterRCNN的图像配准算法研究摘要:图像配准是计算机视觉领域的一个重要研究方向,在医学影像处理、遥感图像处理、工业检测等领域有广泛应用。本论文提出了一种基于FasterRCNN的图像配准算法,通过利用深度学习模型提取特征并进行目标检测,实现了对待配准图像中的目标进行快速准确的定位和匹配,从而提高了配准的精度和效率。实验证明该算法在不同数据集上都取得了良好的配准效果。关键词:图像配准;FasterRCNN;目标检测;深度学习;特征提取一、引言图像配准作为