
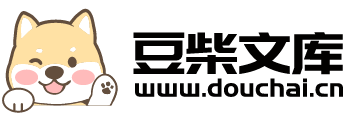
基于CNN和GRU的混合股指预测模型研究.docx
骑着****猪猪
在线预览结束,喜欢就下载吧,查找使用更方便
相关资料
基于CNN和GRU的混合股指预测模型研究.pptx
基于CNN和GRU的混合股指预测模型研究目录添加章节标题引言研究背景研究意义研究目的相关工作CNN在股市预测中的应用GRU在股市预测中的应用混合模型在股市预测中的应用方法论CNN模型构建GRU模型构建混合模型构建数据预处理模型训练与优化实验设计数据集选择实验环境配置实验过程与结果分析模型性能评估指标结果与讨论实验结果展示结果分析与其他模型的比较模型的优缺点分析未来工作展望结论研究成果总结对未来研究的建议THANKYOU
基于CNN和GRU的混合股指预测模型研究.docx
基于CNN和GRU的混合股指预测模型研究AbstractWiththeincreasingimportanceofstockindexforecastinginthefinancialindustry,itiscrucialtodevelopaccurateandefficientpredictionmodels.Inthispaper,weproposeahybridstockindexpredictionmodelbasedonConvolutionalNeuralNetwork(CNN)andGat
基于Adabelief算法的PCA--GRU模型的股指期货预测研究.docx
基于Adabelief算法的PCA--GRU模型的股指期货预测研究基于Adabelief算法的PCA-GRU模型的股指期货预测研究引言:股指期货市场是金融市场中重要的组成部分,对投资者和经济决策者来说具有重要的指导意义。然而,股指期货市场的波动性往往较大,其中涵盖了众多的因素和复杂的相互关系。因此,预测股指期货的价格变动一直是投资者和研究者们关注的热点问题之一。为了能够准确预测股指期货的价格变动,研究者们提出了各种各样的预测模型和算法。其中,基于机器学习的预测模型通常具有较好的预测能力。然而,由于股指期货
融合空间和统计特征的CNN-GRU臭氧浓度预测模型研究.docx
融合空间和统计特征的CNN-GRU臭氧浓度预测模型研究融合空间和统计特征的CNN-GRU臭氧浓度预测模型研究摘要:空气质量是人们关注的热点话题之一,臭氧浓度作为空气质量的重要指标,对人体健康和生态环境有着重要影响。准确地预测臭氧浓度对于采取有效的控制措施、保护健康和环境具有重要意义。本文提出一种融合空间和统计特征的CNN-GRU臭氧浓度预测模型,通过利用卷积神经网络(CNN)提取数据的空间特征,然后将特征输入到门控循环单元(GRU)模型中进行时间序列建模,以实现精准的臭氧浓度预测。通过对实测数据进行实验,
基于AdamW算法的WT--GRU模型在高频股指预测中的应用.docx
基于AdamW算法的WT--GRU模型在高频股指预测中的应用摘要:高频股指预测是金融领域中的重要研究课题。本文以AdamW算法为基础,结合WT--GRU模型,探讨其在高频股指预测中的应用。首先,介绍了AdamW算法和WT--GRU模型的原理和特点。然后,通过案例分析,验证了AdamW算法在优化神经网络中的有效性,以及WT--GRU模型在高频股指预测中的准确性和稳定性。最后,总结了研究的意义和不足,并提出了未来的研究方向。关键词:AdamW算法、WT--GRU模型、高频股指预测引言股指预测一直是金融领域中的