
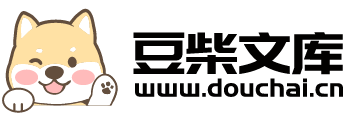
基于TRNSYS与深度学习模型的近零能耗建筑系统能耗与负荷预测.docx
骑着****猪猪
在线预览结束,喜欢就下载吧,查找使用更方便
相关资料
基于TRNSYS与深度学习模型的近零能耗建筑系统能耗与负荷预测.docx
基于TRNSYS与深度学习模型的近零能耗建筑系统能耗与负荷预测AbstractWiththeincreasingdemandforenergy-efficientbuildings,thepredictionofenergyconsumptionandloadofbuildingshasbecomeacriticalissue.Inthispaper,ahybridmodelbasedonTRNSYSanddeeplearningisproposedtopredicttheenergyconsumptio
基于TRNSYS与深度学习模型的近零能耗建筑系统能耗与负荷预测.pptx
汇报人:CONTENTS添加章节标题TRNSYS模型介绍TRNSYS模型的原理TRNSYS模型在建筑能耗预测中的应用TRNSYS模型的优势与局限性深度学习模型介绍深度学习模型的原理深度学习模型在建筑负荷预测中的应用深度学习模型的优势与局限性近零能耗建筑系统能耗与负荷预测方法基于TRNSYS与深度学习模型的能耗与负荷预测流程数据采集与预处理模型训练与优化预测结果分析与评估案例分析案例选择与背景介绍案例实施过程案例结果分析案例总结与启示结论与展望研究结论研究不足与展望汇报人:
近零能耗居住建筑能耗预测方法研究.pptx
近零能耗居住建筑能耗预测方法研究目录近零能耗居住建筑能耗预测方法概述预测方法的定义和重要性预测方法的分类和比较预测方法的应用场景和限制条件近零能耗居住建筑能耗预测方法研究现状国内外研究现状及发展趋势现有预测方法的优缺点分析现有预测方法存在的问题和改进空间近零能耗居住建筑能耗预测方法研究方法研究目标和研究内容研究方法和实验设计数据采集和处理方法近零能耗居住建筑能耗预测方法实现基于数据驱动的预测方法实现基于模型驱动的预测方法实现基于混合驱动的预测方法实现近零能耗居住建筑能耗预测方法评估和比较预测精度评估指标和
近零能耗建筑研究.docx
近零能耗建筑研究1、概念起源及发展HYPERLINK"//www.cbi360.net/"\h1992年,德国Fraunhofer太阳能研究所的Voss.K[1]等人通过使用太阳能光热光电技术对德国一栋建筑物进行供热供暖,并进行了为期三年的检测研究发现:在气候较为温和的欧洲部分地区,通过精心设计可以使建筑物全年总能耗降低到10Kwh/m2以下,且建筑物所有能耗需求可以由太阳能提供。Voss.K由此提出“无源建筑”(EnergyAutonomousHouse,也称Self-sufficientSolar
基于DEST的近零能耗建筑负荷特性研究——以寒冷地区居住建筑为例.docx
基于DEST的近零能耗建筑负荷特性研究——以寒冷地区居住建筑为例基于DEST的近零能耗建筑负荷特性研究——以寒冷地区居住建筑为例摘要:近年来,能源危机和全球气候变化问题严重威胁人类社会的可持续发展。目前,建筑行业占全球能源消耗的40%以上,因此,研究和开发低能耗建筑成为解决能源和环境问题的重要途径。本文针对寒冷地区居住建筑,通过基于DEST(DynamicEnergySimulationTool)的研究方法,对其建筑负荷特性进行了深入研究。通过实验和模拟的方法,分析了在不同季节和气候条件下,建筑中的能耗变