
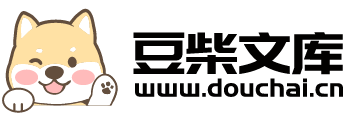
基于AlexNet的雷达干扰识别方法研究.docx
骑着****猪猪
在线预览结束,喜欢就下载吧,查找使用更方便
相关资料
基于AlexNet的雷达干扰识别方法研究.docx
基于AlexNet的雷达干扰识别方法研究AbstractRadarinterferenceisacommonprobleminmodernradarsystems,itcanseverelydegradetheperformanceofradarsystemsandevencausefailureinmission-criticalapplications.Inthispaper,weproposearadarinterferencerecognitionmethodbasedontheAlexNetde
基于改进AlexNet的复杂背景手势识别方法研究.docx
基于改进AlexNet的复杂背景手势识别方法研究摘要本文提出了一种基于改进AlexNet的复杂背景手势识别方法,该方法使用深度学习技术对手势识别任务进行建模。针对复杂背景下的手势识别问题,我们提出了一种数据增强技术来增加数据的多样性和鲁棒性。同时,我们对AlexNet模型进行了改进,将其应用于手势识别任务中。实验结果表明,我们提出的方法具有更高的准确率和更好的鲁棒性,能够更好地应用于复杂背景下的手势识别场景。关键词:深度学习,复杂背景,手势识别,AlexNet模型,数据增强。1.引言手势识别是一种非常重要
基于深度迁移学习的雷达有源干扰信号识别方法.pdf
一种基于深度迁移学习的雷达有源干扰信号识别方法,其步骤包括:1、生成雷达有源干扰信号数据集,2、构建雷达有源干扰信号时频图像训练集、验证集和测试集,3、搭建深度迁移学习网络,4、对深度迁移学习网络进行第一次训练,5、对第一次训练后的深度迁移学习网络进行第二次训练,6、利用深度迁移学习网络预测测试集。本发明能够在雷达有源干扰信号样本数量不充足、不易获取雷达有源干扰信号的条件下实现雷达有源干扰信号的准确分类,加快网络训练时的收敛速度,提高雷达有源干扰信号识别的效率,有利于及时选取有效的抗干扰措施,提升雷达的生
新型雷达干扰识别方法研究的任务书.docx
新型雷达干扰识别方法研究的任务书任务书任务名称:新型雷达干扰识别方法研究任务背景:随着科技的不断发展,军事技术也在不断更新换代。雷达技术作为一种重要的武器装备,其对军事作战具有极为重要的作用。而雷达干扰作为一种常见的战争手段,是对雷达进行干扰、连接、破坏的一种技术手段。如果不能及时准确地识别和应对雷达干扰,将会给军事作战带来重大的威胁和影响。因此,本任务的研究意义重大,将有助于提高我国雷达防护能力,增强国防实力。任务目标:本任务旨在研究一种新型的雷达干扰识别方法,以提高我国雷达防护能力,具体目标如下:1.
基于AlexNet模型的雷达信号调制类型识别.docx
基于AlexNet模型的雷达信号调制类型识别标题:基于AlexNet模型的雷达信号调制类型识别摘要:近年来,雷达技术在通信、无人驾驶、智能制造等领域发挥着重要作用。雷达信号的调制类型识别是雷达系统中的一个重要问题,对于改善信号处理、目标识别和干扰检测等工作具有重要意义。本论文基于AlexNet模型提出了一种雷达信号调制类型识别方法,该方法通过将雷达信号转化为图像,利用深度学习网络实现调制类型的自动识别。实验结果表明,该方法在调制类型识别准确率和性能稳定性方面取得了显著的改进。1.引言雷达信号调制类型识别是